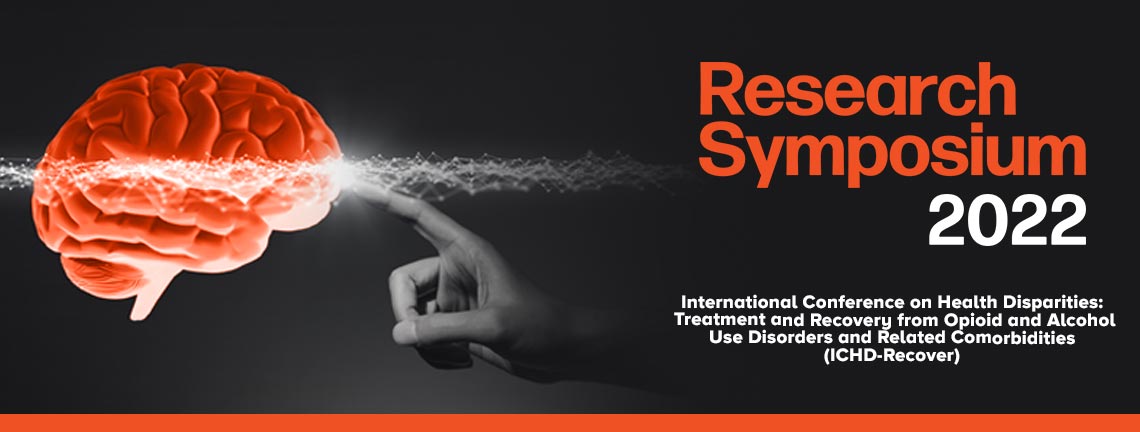
Talks
Presentation Type
Oral Presentation
Discipline Track
Biomedical Science
Abstract Type
Research/Clinical
Abstract
Background: Ambulatory Blood Pressure Measurement (ABPM) allows physicians to monitor blood pressure variability under everyday living conditions and predicts clinical outcomes better than conventional blood-pressure measurement. ABPM can demonstrate mean arterial pressure (MAP) behavior over 24 hours relevant to clinical practice, such as nocturnal hypertension or increased blood pressure variability. We hypothesized that individuals with the same cardiovascular health status would have the same MAP signal (MAPs) waveform.
Methods: This study reutilizes a data subset from the IDACO Consortium to create 24-hour MAPs. We assigned all the MAPs to data matrix X, performed principal components analysis (PCA) to X, and calculated the percentage of the total variance explained by each of the 82 principal components (PC). The first three PC explained 85.03%, 9.47%, and 5.50% of the total variance. We used every MAP signal's first three PC scores as their three-dimensional Euclidean Space (x, y, z) coordinates and assigned them to matrix C. Then, we calculated hierarchical clusters of the rows of C with Ward's linkage minimum variance algorithm and a Euclidian metric and encoded this information on the agglomerative hierarchical cluster tree Z. We determined the gap statistic in Z to obtain the optimal number of clusters. We created seven agglomerative clusters from the linkages stored in Z, using Ward’s distance as the criterion for defining the clusters. Finally, we plotted and colored the mapped MAPs by their assigned cluster number at the locations specified by their (x, y, z) coordinates.
Results: The MAPs cartesian representations show that MAPs with similar waveforms cluster in the same three-dimensional Euclidean subspace. These patterns identified individuals with dipping and non-dipping blood pressure behavior, which is relevant to clinical management.
Conclusions: Mapping a set of physiological signals into a Euclidian space creates a mathematical formalism that provides a statistical framework to classify physiological signals by their waveform. By applying our method to existing electrophysiological and physiological databases, we can cluster any biomedical time-series (blood pressure, ECG, EEG, EMG, patch-clamp, single-unit recordings, etc.) by physiologic or pathological waveform, so further epidemiological and genetic studies can be conducted on the subjects or tissue samples sharing similar patterns.
Academic/Professional Position
Post-doc
Mentor/PI Department
Office of Human Genetics
Recommended Citation
Garcia, Antonio; Maestre, Gladys E.; Blangero, John; Melgarejo, Jesus D.; and Facelli, Julio, "Using Cartesian Coordinate Systems to Create, Classify, and Retrieve Biomedical Time-Series: Applications to 24-hour Ambulatory Blood Pressure Monitoring" (2023). Research Symposium. 9.
https://scholarworks.utrgv.edu/somrs/2022/talks/9
Included in
Cardiovascular Diseases Commons, Diagnosis Commons, Nervous System Diseases Commons, Other Analytical, Diagnostic and Therapeutic Techniques and Equipment Commons
Using Cartesian Coordinate Systems to Create, Classify, and Retrieve Biomedical Time-Series: Applications to 24-hour Ambulatory Blood Pressure Monitoring
Background: Ambulatory Blood Pressure Measurement (ABPM) allows physicians to monitor blood pressure variability under everyday living conditions and predicts clinical outcomes better than conventional blood-pressure measurement. ABPM can demonstrate mean arterial pressure (MAP) behavior over 24 hours relevant to clinical practice, such as nocturnal hypertension or increased blood pressure variability. We hypothesized that individuals with the same cardiovascular health status would have the same MAP signal (MAPs) waveform.
Methods: This study reutilizes a data subset from the IDACO Consortium to create 24-hour MAPs. We assigned all the MAPs to data matrix X, performed principal components analysis (PCA) to X, and calculated the percentage of the total variance explained by each of the 82 principal components (PC). The first three PC explained 85.03%, 9.47%, and 5.50% of the total variance. We used every MAP signal's first three PC scores as their three-dimensional Euclidean Space (x, y, z) coordinates and assigned them to matrix C. Then, we calculated hierarchical clusters of the rows of C with Ward's linkage minimum variance algorithm and a Euclidian metric and encoded this information on the agglomerative hierarchical cluster tree Z. We determined the gap statistic in Z to obtain the optimal number of clusters. We created seven agglomerative clusters from the linkages stored in Z, using Ward’s distance as the criterion for defining the clusters. Finally, we plotted and colored the mapped MAPs by their assigned cluster number at the locations specified by their (x, y, z) coordinates.
Results: The MAPs cartesian representations show that MAPs with similar waveforms cluster in the same three-dimensional Euclidean subspace. These patterns identified individuals with dipping and non-dipping blood pressure behavior, which is relevant to clinical management.
Conclusions: Mapping a set of physiological signals into a Euclidian space creates a mathematical formalism that provides a statistical framework to classify physiological signals by their waveform. By applying our method to existing electrophysiological and physiological databases, we can cluster any biomedical time-series (blood pressure, ECG, EEG, EMG, patch-clamp, single-unit recordings, etc.) by physiologic or pathological waveform, so further epidemiological and genetic studies can be conducted on the subjects or tissue samples sharing similar patterns.