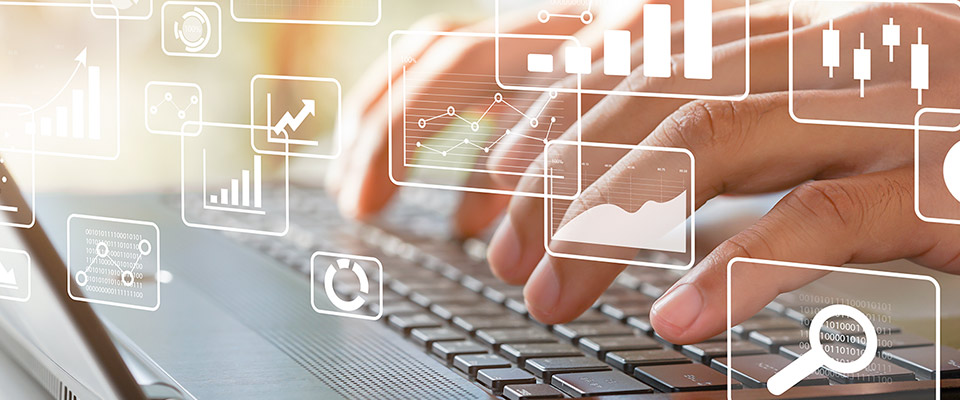
School of Mathematical and Statistical Sciences Faculty Publications and Presentations
Turbulence compensation for radar imaging carrying orbital angular momentum based on convolutional neural network
Document Type
Article
Publication Date
2-24-2023
Abstract
The spiral phase front (SPF) and orbital angular momentum (OAM) of vortex electromagnetic waves (VEMW) have attracted extensive attention in radar imaging. However, VEMW is extremely sensitive to atmospheric turbulence (AT), which will lead to distortion of the SPF and diffusion of the OAM modes. Furthermore, refractive-index fluctuations will cause absorption and fluctuations in the speed of VEMW. Specifically, SPF distortion leads to phase errors, which is demonstrated as defocus of the scatterer in the image. Atmospheric absorption and fluctuations in VEMW speed suffer amplitude error and phase error, which are manifested as amplitude variation with range and positioning errors, respectively. Generally, refractive-index fluctuations feature significant spatial and temporal variability, making it difficult for conventional algorithms to obtain accurate compensation. In this paper, a data-driven-based convolutional neural network (CNN) approach is proposed to improve imaging performance. The CNN is taken to learn the mapping function of images with atmospheric errors to label images without turbulence effects, and then the trained CNN model is integrated into imaging system to improve the imaging quality. The numerical simulation verifies that the method can effectively compensate for turbulence effects and obtain high-resolution images.
Recommended Citation
Jianwen Guo, Hongyin Shi, Ting Yang & Zhijun Qiao (2023) Turbulence compensation for radar imaging carrying orbital angular momentum based on convolutional neural network, Waves in Random and Complex Media, DOI: 10.1080/17455030.2023.2181644
Publication Title
Waves in Random and Complex Media
DOI
10.1080/17455030.2023.2181644
Comments
https://www.tandfonline.com/share/8FIRCWSUPHVJSXRZENQX?target=10.1080/17455030.2023.2181644