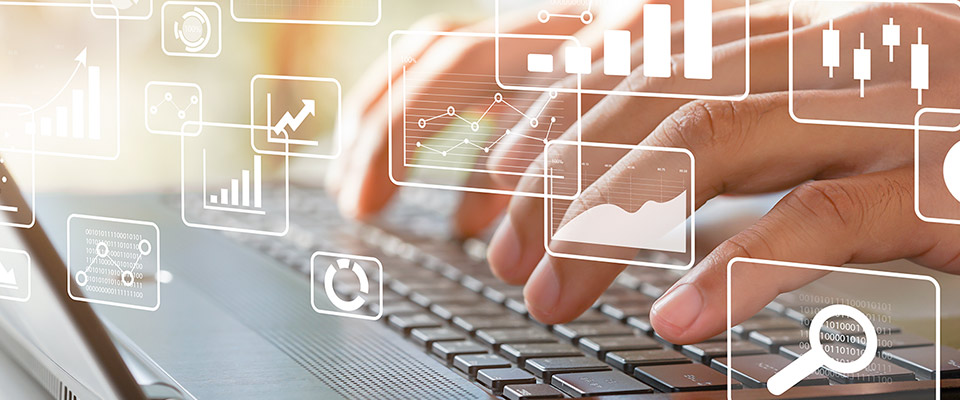
School of Mathematical and Statistical Sciences Faculty Publications and Presentations
Document Type
Article
Publication Date
10-12-2023
Abstract
Some individuals complain of listening-in-noise difficulty despite having a normal audiogram. In this study, machine learning is applied to examine the extent to which hearing thresholds can predict speech-in-noise recognition among normal-hearing individuals. The specific goals were to (1) compare the performance of one standard (GAM, generalized additive model) and four machine learning models (ANN, artificial neural network; DNN, deep neural network; RF, random forest; XGBoost; eXtreme gradient boosting), and (2) examine the relative contribution of individual audiometric frequencies and demographic variables in predicting speech-in-noise recognition. Archival data included thresholds (0.25–16 kHz) and speech recognition thresholds (SRTs) from listeners with clinically normal audiograms (n = 764 participants or 1528 ears; age, 4–38 years old). Among the machine learning models, XGBoost performed significantly better than other methods (mean absolute error; MAE = 1.62 dB). ANN and RF yielded similar performances (MAE = 1.68 and 1.67 dB, respectively), whereas, surprisingly, DNN showed relatively poorer performance (MAE = 1.94 dB). The MAE for GAM was 1.61 dB. SHapley Additive exPlanations revealed that age, thresholds at 16 kHz, 12.5 kHz, etc., on the order of importance, contributed to SRT. These results suggest the importance of hearing in the extended high frequencies for predicting speech-in-noise recognition in listeners with normal audiograms.
Recommended Citation
Balan, J. R., Rodrigo, H., Saxena, U., & Mishra, S. K. (2023). Explainable machine learning reveals the relationship between hearing thresholds and speech-in-noise recognition in listeners with normal audiograms. The Journal of the Acoustical Society of America, 154(4), 2278-2288. https://doi.org/10.1121/10.0021303
Publication Title
The Journal of the Acoustical Society of America
DOI
10.1121/10.0021303
Comments
© 2023 Acoustical Society of America. Original published version available at https://doi.org/10.1121/10.0021303