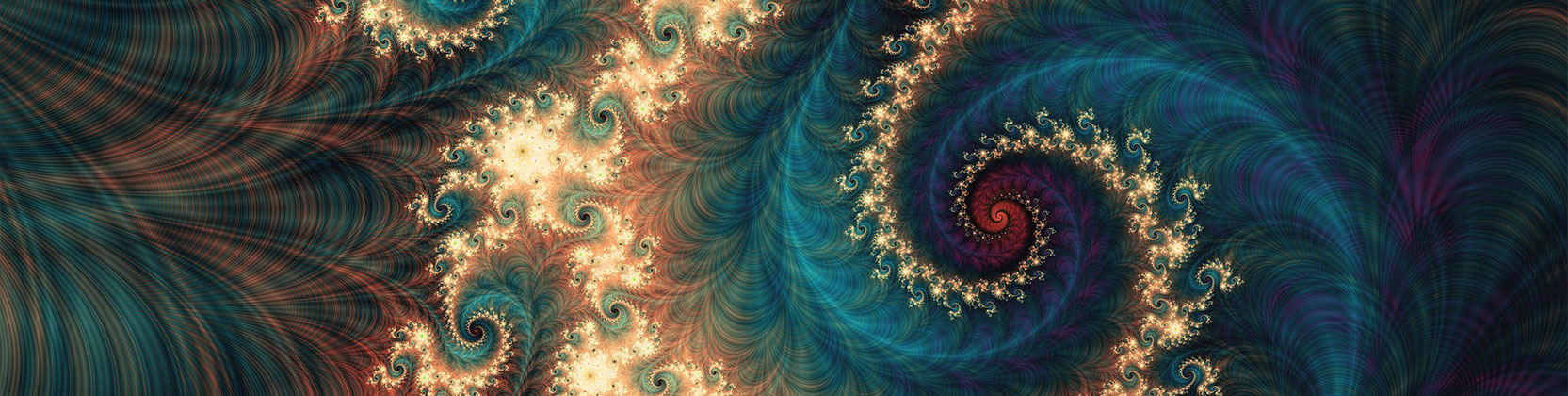
School of Mathematical and Statistical Sciences Faculty Publications and Presentations
Document Type
Article
Publication Date
9-30-2024
Abstract
Predicting academic outcomes is complex and influenced by factors like socioeconomic background, motivation, and learning style. Machine Learning (ML) algorithms have become increasingly important due to their ability to analyze large data volumes and identify prediction patterns. Results show ML’s success in predicting academic performance, though effectiveness varies by dataset, algorithm choice, and training features. There is no consensus on the most effective ML method for predicting student performance with broad applicability. Among the five algorithms evaluated in this study, the Random Forest Classifier emerged as the best model, achieving the highest G-Mean and accuracy of 0.9243 and 85.42% respectively. This model's performance emphasizes the importance of balanced sensitivity and specificity in predicting student academic performance. The empirical review highlights several challenges, including a lack of standardization in performance metrics, limited model generalizability, and potential bias in training data. It also notes the impact of individual and environmental factors on academic performance, emphasizing the role of instructors and policymakers in improving educational outcomes. The study provides insights into current trends in using ML algorithms for academic predictions, identifying conceptual, methodological, analytical, and ethical gaps. These gaps affect the validity and reliability of research, underscoring the need to address them for informed decision-making and improved learning outcomes.
Recommended Citation
Agyemang, Edmund F., Joseph A. Mensah, Obu-Amoah Ampomah, Louis Agyekum, Justice Akuoko-Frimpong, Amma Quansah, and Oluwaferanmi M. Akinlosotu. 2024. “Predicting Students’ Academic Performance Via Machine Learning Algorithms: An Empirical Review and Practical Application.” Computer Engineering and Intelligent Systems 15 (1): 86–102. http://doi.org/10.7176/CEIS/15-1-09
Creative Commons License
This work is licensed under a Creative Commons Attribution 3.0 License.
First Page
86
Last Page
102
Publication Title
Computer Engineering and Intelligent Systems
DOI
http://doi.org/10.7176/CEIS/15-1-09
Comments
Student publication.
The journal is licensed under a Creative Commons Attribution 3.0 Unported (CC BY 3.0) License.