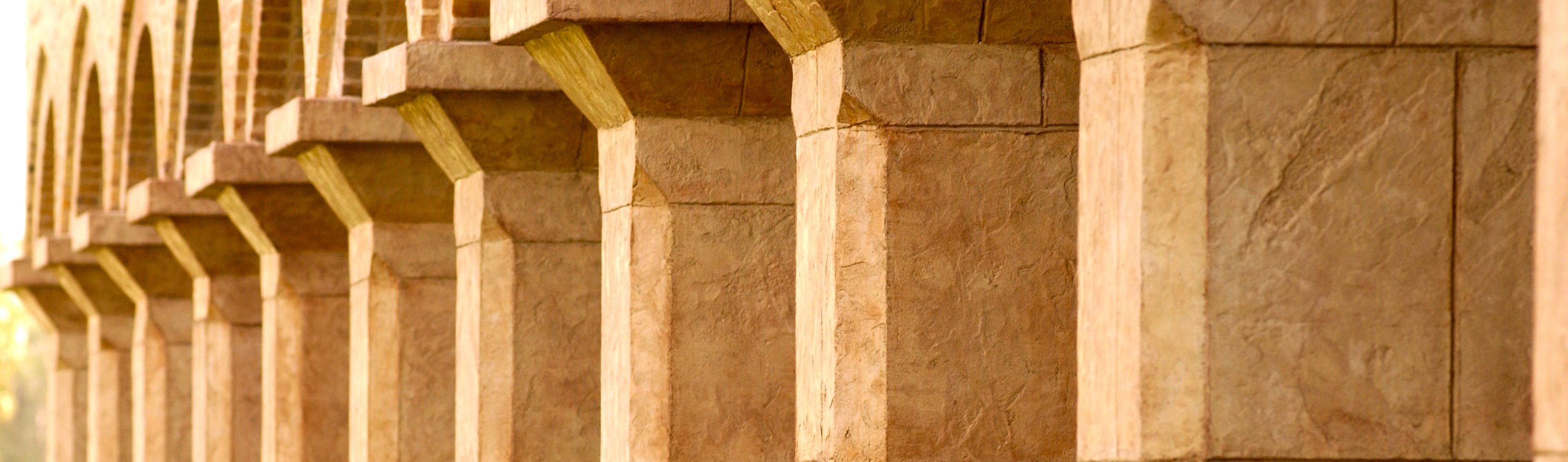
Civil Engineering Faculty Publications and Presentations
Document Type
Article
Publication Date
12-10-2024
Abstract
The evaluation of instantaneous wind flow patterns nearest to building architecture is crucial to ensuring structural stability, architectural integrity, and pedestrian safety. Particle image velocimetry (PIV), a technique for studying fluid flow by tracing particles, provides accurate predictions of instantaneous wind velocities (IWV). However, PIV encounters challenges in specific regions due to laser light-based experimentation, leading to missing data. Consequently, investigating the wind circulation pattern around buildings becomes more challenging. Numerous ML techniques have been employed to impute missing wind velocities at random building locations with minimal structural impact. This paper focuses on addressing this concern by utilizing a machine learning (ML) approach that focuses on estimating unmeasured values in critical areas near buildings. We employ three distinct ML models: the generative adversarial imputation network (GAIN), multiple imputations by chained equations (MICE), and neighbor distance imputation (NDI) to estimate missing values around building structures. Our results indicate that the GAIN technique achieves a remarkable balance, displaying the lowest average mean square error of 0.073 and the highest average R-squared error of 0.965. Furthermore, it effectively captures the distribution of measured values and provides reliable data for evaluating aerodynamic characteristics and ensuring structural safety.
Recommended Citation
Ahammed, Istiak, Sujeen Song, Gang Hu, Jinwoo An, and Bubryur Kim. "Enhanced wind velocity imputation near building structures using advanced machine learning techniques." Wind and Structures 39, no. 6 (2024): 435-451. https://doi.org/10.12989/was.2024.39.6.435
First Page
435
Last Page
451
Publication Title
Wind and Structures
DOI
10.12989/was.2024.39.6.435
Comments
Copyright © 2024 Techno-Press, Ltd. Posted with permission from publisher.
KoreaScience