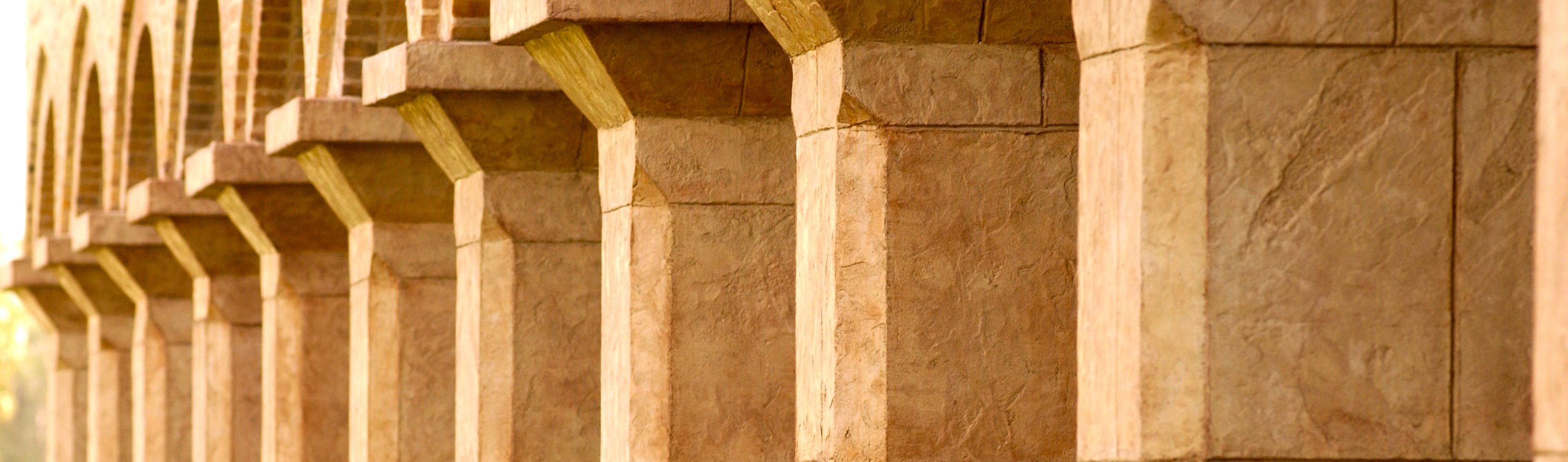
Civil Engineering Faculty Publications and Presentations
Document Type
Article
Publication Date
3-15-2025
Abstract
Accurate forecasting of wind speed and direction is critical for the efficient integration of wind power into energy systems, ensuring reliable renewable energy production and grid stability. Traditional methods often struggle with capturing nonlinear interdependencies, quantifying uncertainties, and providing reliable long-term predictions, particularly in complex atmospheric conditions. To address these challenges, this study introduces multi-model Integration for dynamic forecasting (MIDF), an ensemble machine learning framework that combines the strengths of DeepAR and temporal fusion transformer (TFT) models through a two-step meta-learning process. MIDF leverages DeepAR’s probabilistic forecasting capabilities and TFT’s attention mechanisms to enhance accuracy, robustness, and interpretability. Using a custom meteorological dataset spanning January 2010 to May 2023, the model was evaluated against standalone alternatives across multiple metrics, including MSE, RMSE, and R2. MIDF achieved superior performance, with MSE, RMSE, and R2 values of 0.0035, 0.01913, and 0.89 for wind speed, and 0.00052, 0.02507, and 0.86 for wind direction, significantly reducing errors compared to existing methods. These results underscore the potential of ensemble learning in advancing wind forecasting accuracy, enabling more reliable renewable energy management, operational planning, and risk mitigation in meteorological applications.
Recommended Citation
Maruthi, Molaka, Bubryur Kim, Song Sujeen, Jinwoo An, and Zengshun Chen. "Multi-model integration for dynamic forecasting (MIDF): a framework for wind speed and direction prediction." Artificial Intelligence Review 58, no. 6 (2025): 164. https://doi.org/10.1007/s10462-025-11140-x
Creative Commons License
This work is licensed under a Creative Commons Attribution-NonCommercial-No Derivative Works 4.0 International License.
Publication Title
Artificial Intelligence Review
DOI
10.1007/s10462-025-11140-x
Comments
This article is licensed under a Creative Commons Attribution-NonCommercial-NoDerivatives 4.0 International License, which permits any non-commercial use, sharing, distribution and reproduction in any medium or format, as long as you give appropriate credit to the original author(s) and the source, provide a link to the Creative Commons licence, and indicate if you modified the licensed material.