Document Type
Article
Publication Date
11-2023
Abstract
Highlights
-
Major traditional and deep learning methods on brain network representation are overviewed.
-
Brain network datasets and algorithm implementation tools are summarized.
-
Promising research directions in brain network analysis are discussed.
Abstract
Recent years have shown great merits in utilizing neuroimaging data to understand brain structural and functional changes, as well as its relationship to different neurodegenerative diseases and other clinical phenotypes. Brain networks, derived from different neuroimaging modalities, have attracted increasing attention due to their potential to gain system-level insights to characterize brain dynamics and abnormalities in neurological conditions. Traditional methods aim to pre-define multiple topological features of brain networks and relate these features to different clinical measures or demographical variables. With the enormous successes in deep learning techniques, graph learning methods have played significant roles in brain network analysis. In this survey, we first provide a brief overview of neuroimaging-derived brain networks. Then, we focus on presenting a comprehensive overview of both traditional methods and state-of-the-art deep-learning methods for brain network mining. Major models, and objectives of these methods are reviewed within this paper. Finally, we discuss several promising research directions in this field.
Recommended Citation
Tang, Haoteng, Guixiang Ma, Yanfu Zhang, Kai Ye, Lei Guo, Guodong Liu, Qi Huang et al. "A Comprehensive Survey of Complex Brain Network Representation." Meta-Radiology (2023): 100046. https://doi.org/10.1016/j.metrad.2023.100046
Creative Commons License
This work is licensed under a Creative Commons Attribution-NonCommercial-No Derivative Works 4.0 International License.
Publication Title
Meta-Radiology
DOI
10.1016/j.metrad.2023.100046
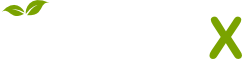
- Citations
- Citation Indexes: 11
- Usage
- Downloads: 65
- Abstract Views: 7
- Captures
- Readers: 16
- Mentions
- News Mentions: 1
Comments
Under a Creative Commons license