Document Type
Article
Publication Date
1-2020
Abstract
It’s critical for an autonomous vehicle to acquire accurate and real-time information of the objects in its vicinity, which will fully guarantee the safety of the passengers and vehicle in various environment. 3D LIDAR can directly obtain the position and geometrical structure of the object within its detection range, while vision camera is very suitable for object recognition. Accordingly, this paper presents a novel object detection and identification method fusing the complementary information of two kind of sensors. We first utilize the 3D LIDAR data to generate accurate object-region proposals effectively. Then, these candidates are mapped into the image space where the regions of interest (ROI) of the proposals are selected and input to a convolutional neural network (CNN) for further object recognition. In order to identify all sizes of objects precisely, we combine the features of the last three layers of the CNN to extract multi-scale features of the ROIs. The evaluation results on the KITTI dataset demonstrate that : (1) Unlike sliding windows that produce thousands of candidate object-region proposals, 3D LIDAR provides an average of 86 real candidates per frame and the minimal recall rate is higher than 95%, which greatly lowers the proposals extraction time; (2) The average processing time for each frame of the proposed method is only 66.79ms, which meets the real-time demand of autonomous vehicles; (3) The average identification accuracies of our method for car and pedestrian on the moderate level are 89.04% and 78.18% respectively, which outperform most previous methods.
Recommended Citation
Zhao, X., Sun, P., Xu, Z., Min, H., & Yu, H. (2020). Fusion of 3D LIDAR and Camera Data for Object Detection in Autonomous Vehicle Applications. Ieee Sensors Journal, 20(9), 4901–4913. https://doi.org/10.1109/JSEN.2020.2966034
Publication Title
IEEE Sensors Journal
DOI
10.1109/JSEN.2020.2966034
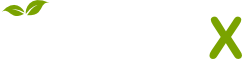
- Citations
- Citation Indexes: 256
- Patent Family Citations: 7
- Policy Citations: 1
- Usage
- Downloads: 5798
- Abstract Views: 136
- Captures
- Readers: 271
Comments
© 2019 IEEE. Original published version available at https://doi.org/10.1109/JSEN.2020.2966034