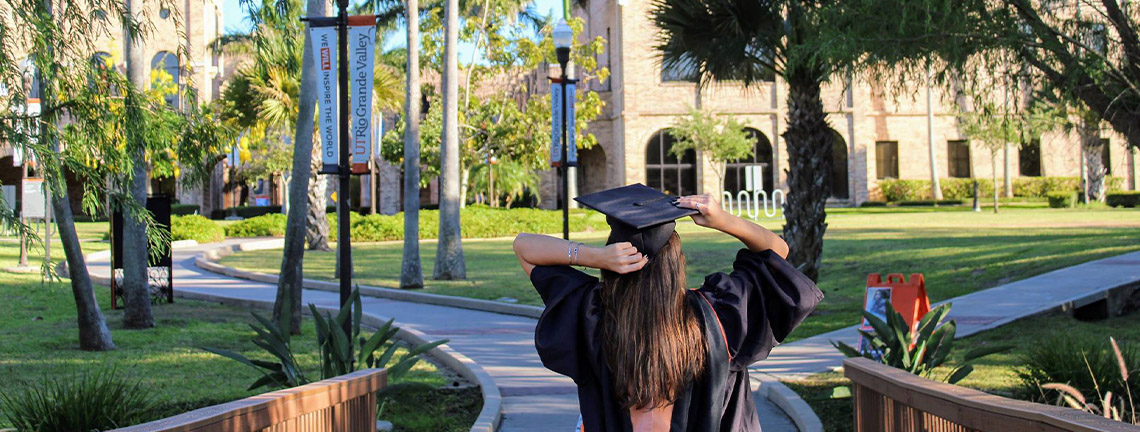
Theses and Dissertations
Date of Award
7-2023
Document Type
Thesis
Degree Name
Master of Science (MS)
Department
Applied Statistics and Data Science
First Advisor
Hansapani Rodrigo
Second Advisor
Tamer Oraby
Third Advisor
George Yanev
Abstract
Breast cancer is the second most prevalent form of cancer in women in the United States. Each year, about 264,000 cases of breast cancer are diagnosed in women and of this number, about 42,000 women lose their lives as reported by the Centers for Disease Control and Prevention. Early detection and effective treatment are crucial for improving survival rates and reducing mortality. This study aimed to explore the influential factors that may risk the survival of women with the disease and compare their predictive abilities using several error and performance metrics. The study uses a dataset from the National Cancer Institute's Surveillance, Epidemiology, and End Results program containing information on 4024 women with infiltrating duct and lobular carcinoma breast cancer diagnosed between 2006 - 2010. We adopt the ensemble technique, Random Survival Forest which was built as a time-to-event extension of the random forest that can handle high-dimensional data and interactions between variables, and the Cox Proportional Deep Neural Network which can handle complex nonlinear relationships between covariates. The LASSO Cox regression technique was employed as a variable selection method to be used in building the models. To improve the interpretability of the results, the Shapley Additive explanation was utilized in the study to shed light on the models' performance and to facilitate the interpretation of the model's variables, using the features obtained from the Cox regression hazard model and Machine Learning techniques such as the Extreme Gradient Boosting, LightGBM, SVM with RBF Kernel and Random Forests algorithms as a benchmark.
Recommended Citation
Baidoo, Theophilus Gyedu, "Advanced Prognostic Modeling for Breast Cancer Patients: Leveraging Data-Driven Approaches for Survival Analysis" (2023). Theses and Dissertations. 1281.
https://scholarworks.utrgv.edu/etd/1281
Comments
Copyright 2023 Theophilus Gyedu Baidoo. All Rights Reserved.
https://go.openathens.net/redirector/utrgv.edu?url=https://www.proquest.com/dissertations-theses/advanced-prognostic-modeling-breast-cancer/docview/2861645881/se-2?accountid=7119