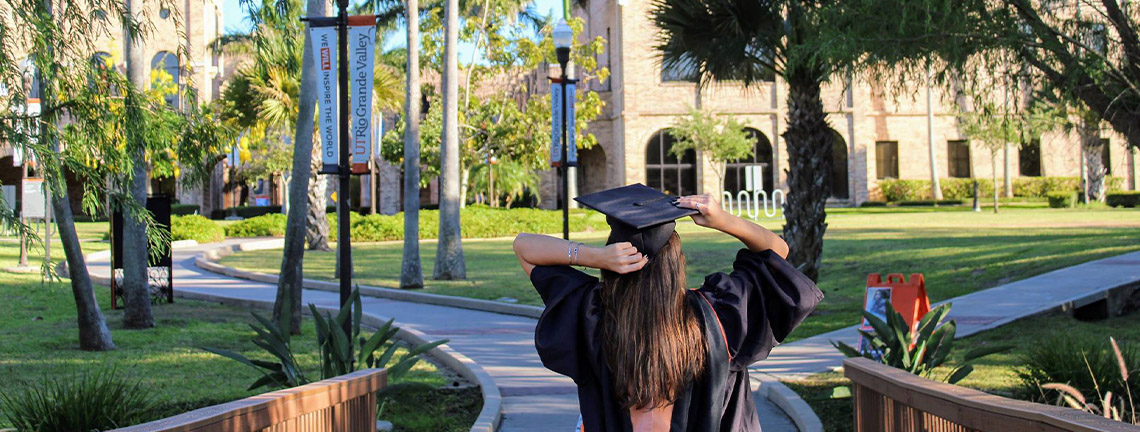
Theses and Dissertations
Date of Award
12-2019
Document Type
Thesis
Degree Name
Master of Science (MS)
Department
Computer Science
First Advisor
Dr. Hongkai Yu
Second Advisor
Dr. Jingru Zhang
Third Advisor
Dr. Emmett Tomai
Abstract
With the wide use of smart phones, distraction has become a major safety concern to roadway users. The distracted phone-use behaviors among pedestrians, like Texting, Game Playing and Phone Calls, have caused increasing fatalities and serious injuries. With the increasing usage of driver monitor systems on intelligent vehicles, distracted driver behaviors can be efficiently detected and warned. However, the research of phone-related distracted behavior by pedestrians has not been systemically studied. It is desired to improve both the driving and pedestrian safety by automatically discovering the phone-related pedestrian distracted behaviors. In this thesis, we propose a new computer vision-based method to detect the phone-related pedestrian distracted behaviors from a view of intelligent and autonomous driving. Specifically, we design the first end-to-end deep learning based Two-Branch Convolutional Neural Network (CNN) for this task. Taking one synchronized image pair by two front on-car GoPro cameras as the inputs, the proposed two-branch CNN will extract features for each camera, fuse the extracted features and perform a robust classification. This method can also be easily extended to video-based classification by confidence accumulation and voting. We propose a new benchmark dataset of 448 synchronized video pairs of 53,760 images collected on a vehicle for this research problem, where the proposed method achieved an overall best classification accuracy of 84.3% on the new benchmark when compared to other methods.
Recommended Citation
Saenz, Humberto, "Detecting Phone-Related Pedestrian Behavior Using a Two-Branch Convolutional Neural Network" (2019). Theses and Dissertations. 578.
https://scholarworks.utrgv.edu/etd/578
Comments
Copyright 2019 Humberto Saenz. All Rights Reserved.
https://go.openathens.net/redirector/utrgv.edu?url=https://www.proquest.com/dissertations-theses/detecting-phone-related-pedestrian-behavior-using/docview/2370530307/se-2?accountid=7119