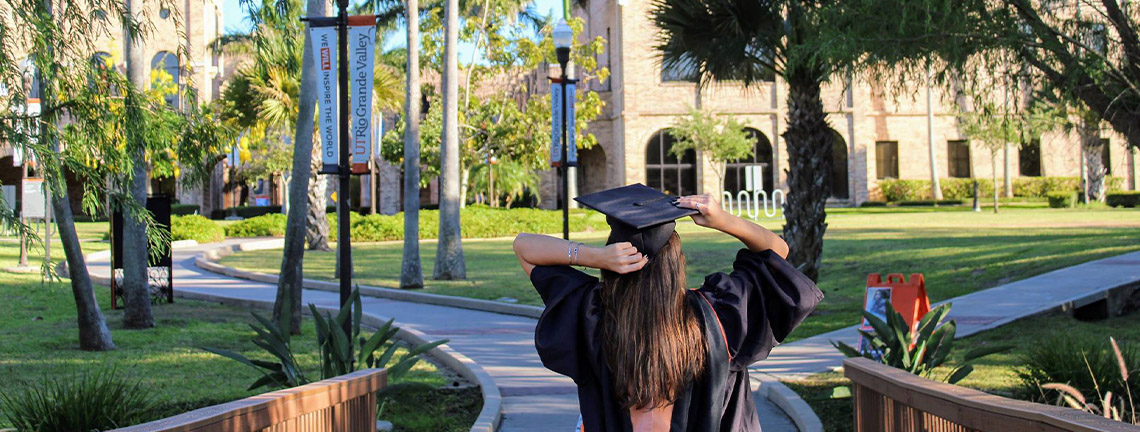
Theses and Dissertations
Date of Award
8-2020
Document Type
Dissertation
Degree Name
Doctor of Philosophy (PhD)
Department
Information Systems
First Advisor
Dr. Bin Wang
Second Advisor
Dr. Diego Escobari
Third Advisor
Dr. Jun Sun
Abstract
Misinformation has captured the interest of academia in recent years with several studies looking at the topic broadly. However, these studies mostly focused on rumors which are social in nature and can be either classified as false or real. In this research, we attempt to bridge the gap in the literature by examining the impacts of user characteristics and feature contents on the diffusion of (mis)information using verified true and false information. We apply a topic allocation model augmented by both supervised and unsupervised machine learning algorithms to identify tweets on novel topics. We find that retweet count is higher for fake news, novel tweets, and tweets with negative sentiment and lower lexical structure. In addition, our results show that the impacts of sentiment are opposite for fake news versus real news. We also find that tweets on the environment have a lower retweet count than the baseline religious news and real social news tweets are shared more often than fake social news. Furthermore, our studies show the counter intuitive nature of current correction endeavors by FEMA and other fact checking organizations in combating falsehoods. Specifically, we show that even though fake news causes an increase in correction messages, they influenced the propagation of falsehoods. Finally our empirical results reveal that correction messages, positive tweets and emotionally charged tweets morph faster. Furthermore, we show that tweets with positive sentiment or are emotionally charged morph faster over time. Word count and past morphing history also positively affect morphing behavior.
Recommended Citation
King, Kelvin Kizito, "Diffusion of Falsehoods on Social Media" (2020). Theses and Dissertations. 689.
https://scholarworks.utrgv.edu/etd/689
Comments
Copyright 2020 Kelvin K. King. All Rights Reserved.
https://go.openathens.net/redirector/utrgv.edu?url=https://www.proquest.com/dissertations-theses/diffusion-falsehoods-on-social-media/docview/2502246446/se-2?accountid=7119