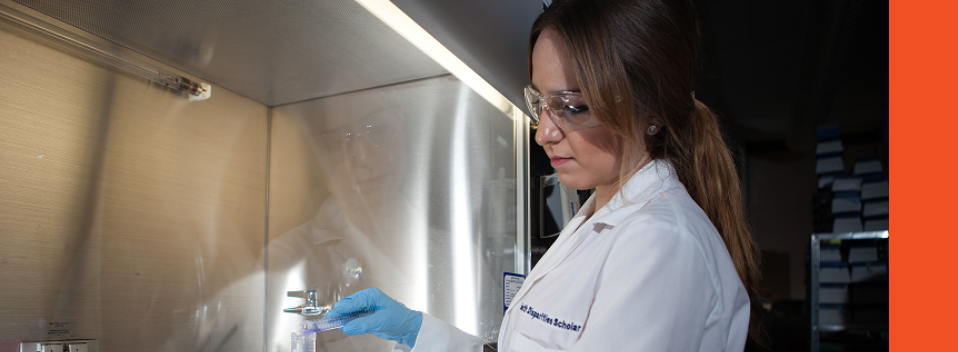
Health & Biomedical Sciences Faculty Publications and Presentations
Document Type
Article
Publication Date
10-15-2023
Abstract
Objective
Metabolic biomarkers can potentially inform disease progression in Alzheimer's disease (AD). The purpose of this study is to identify and describe a new set of diagnostic biomarkers for developing deep learning (DL) tools to predict AD using Ultra Performance Liquid Chromatography Mass Spectrometry (UPLC-MS/MS)-based metabolomicsdata.
Methods
A total of 177 individuals, including 78 with AD and 99 with cognitive normal (CN), were selected from the Alzheimer's Disease Neuroimaging Initiative (ADNI) cohort along with 150 metabolomic biomarkers. We performed feature selection using the Least Absolute Shrinkage and Selection Operator (LASSO). The H2O DL function was used to build multilayer feedforward neural networks to predict AD.
Results
The LASSO selected 21 metabolic biomarkers. To develop DL models, the 21 biomarkers identified by LASSO were imported into the H2O package. The data was split into 70% for training and 30% for validation. The best DL model with two layers and 18 neurons achieved an accuracy of 0.881, F1-score of 0.892, and AUC of 0.873. Several metabolomic biomarkers involved in glucose and lipid metabolism, in particular bile acid metabolites, were associated with APOE-ε4 allele and clinical biomarkers (Aβ42, tTau, pTau), cognitive assessments [the Alzheimer's Disease Assessment Scale-cognitive subscale 13 (ADAS13), the Mini-Mental State Examination (MMSE)], and hippocampus volume.
Conclusions
This study identified a new set of diagnostic metabolomic biomarkers for developing DL tools to predict AD. These biomarkers may help with early diagnosis, prognostic risk stratification, and/or early treatment interventions for patients at risk for AD.
Recommended Citation
Wang, K., Theeke, L. A., Liao, C., Wang, N., Lu, Y., Xiao, D., ... & Alzheimer's Disease Neuroimaging Initiative. (2023). Deep learning analysis of UPLC-MS/MS-based metabolomics data to predict Alzheimer's disease. Journal of the neurological sciences, 453, 120812. https://doi.org/10.1016/j.jns.2023.120812
Creative Commons License
This work is licensed under a Creative Commons Attribution-NonCommercial-No Derivative Works 4.0 International License.
Publication Title
Journal of the Neurological Sciences
DOI
https://doi.org/10.1016/j.jns.2023.120812
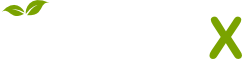
- Citations
- Citation Indexes: 6
- Usage
- Downloads: 22
- Abstract Views: 12
- Captures
- Readers: 23
- Mentions
- News Mentions: 7
Comments
Original published version available at https://doi.org/10.1016/j.jns.2023.120812