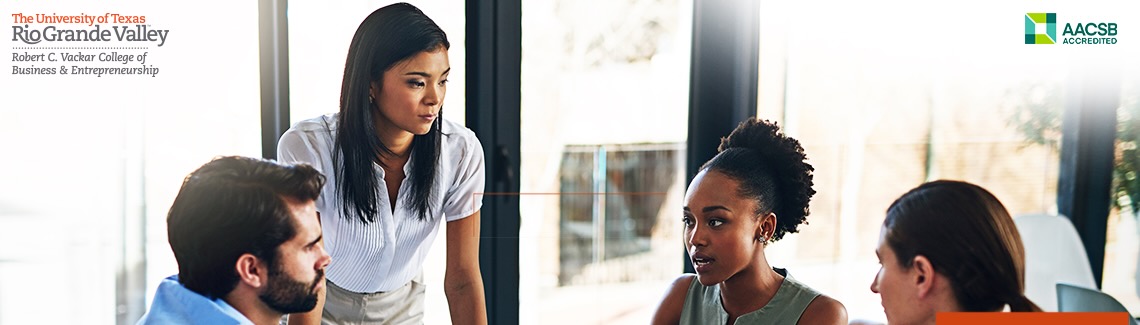
Information Systems Faculty Publications and Presentations
Document Type
Article
Publication Date
6-2021
Abstract
With the development of information and communication technologies, all public tertiary hospitals in China began to use online outpatient appointment systems. However, the phenomenon of patient no-shows in online outpatient appointments is becoming more serious. The objective of this study is to design a prediction model for patient no-shows, thereby assisting hospitals in making relevant decisions, and reducing the probability of patient no-show behavior. We used 382,004 original online outpatient appointment records, and divided the data set into a training set (N1 = 286,503), and a validation set (N2 = 95,501). We used machine learning algorithms such as logistic regression, k-nearest neighbor (KNN), boosting, decision tree (DT), random forest (RF) and bagging to design prediction models for patient no-show in online outpatient appointments. The patient no-show rate of online outpatient appointment was 11.1% (N = 42,224). From the validation set, bagging had the highest area under the ROC curve and AUC value, which was 0.990, followed by random forest and boosting models, which were 0.987 and 0.976, respectively. In contrast, compared with the previous prediction models, the area under ROC and AUC values of the logistic regression, decision tree, and k-nearest neighbors were lower at 0.597, 0.499 and 0.843, respectively. This study demonstrates the possibility of using data from multiple sources to predict patient no-shows. The prediction model results can provide decision basis for hospitals to reduce medical resource waste, develop effective outpatient appointment policies, and optimize operations.
Recommended Citation
Fan, Guorui, et al. "Machine learning-based prediction models for patients no-show in online outpatient appointments." Data Science and Management 2 (2021): 45-52. https://doi.org/10.1016/j.dsm.2021.06.002
Creative Commons License
This work is licensed under a Creative Commons Attribution-NonCommercial-No Derivative Works 4.0 International License.
Publication Title
Data Science and Management
DOI
10.1016/j.dsm.2021.06.002
Comments
© 2021 Xi'an Jiaotong University. Publishing services by Elsevier B.V. on behalf of KeAi Communications Co. Ltd.