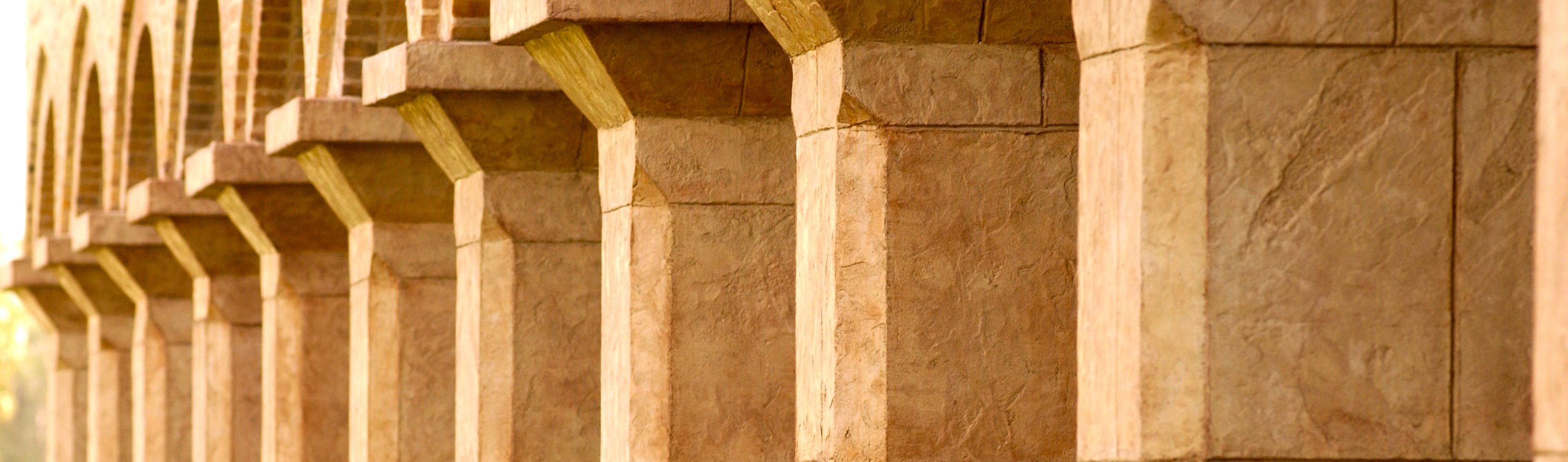
Manufacturing & Industrial Engineering Faculty Publications and Presentations
Document Type
Conference Proceeding
Publication Date
11-3-2021
Abstract
Digital twin is a vital enabling technology for smart manufacturing in the era of Industry 4.0. Digital twin effectively replicates its physical asset enabling easy visualization, smart decision-making and cognitive capability in the system. In this paper, a framework of dynamic data driven digital twin for complex engineering products was proposed. To illustrate the proposed framework, an example of health management on aircraft engines was studied. This framework models the digital twin by extracting information from the various sensors and Industry Internet of Things (IIoT) monitoring the remaining useful life (RUL) of an engine in both cyber and physical domains. Then, with sensor measurements selected from linear degradation models, a long short-term memory (LSTM) neural network is proposed to dynamically update the digital twin, which can estimate the most up-to-date RUL of the physical aircraft engine. Through comparison with other machine learning algorithms, including similarity based linear regression and feed forward neural network, on RUL modelling, this LSTM based dynamical data driven digital twin provides a promising tool to accurately replicate the health status of aircraft engines. This digital twin based RUL technique can also be extended for health management and remote operation of manufacturing systems.
Recommended Citation
Wu, Zhenhua, and Jianzhi Li. "A Framework of Dynamic Data Driven Digital Twin for Complex Engineering Products: the Example of Aircraft Engine Health Management." Procedia Manufacturing 55 (2021): 139-146. https://doi.org/10.1016/j.promfg.2021.10.020
Creative Commons License
This work is licensed under a Creative Commons Attribution-NonCommercial-No Derivative Works 4.0 International License.
DOI
10.1016/j.promfg.2021.10.020
Comments
© 2021 The Author(s). Published by Elsevier B.V.