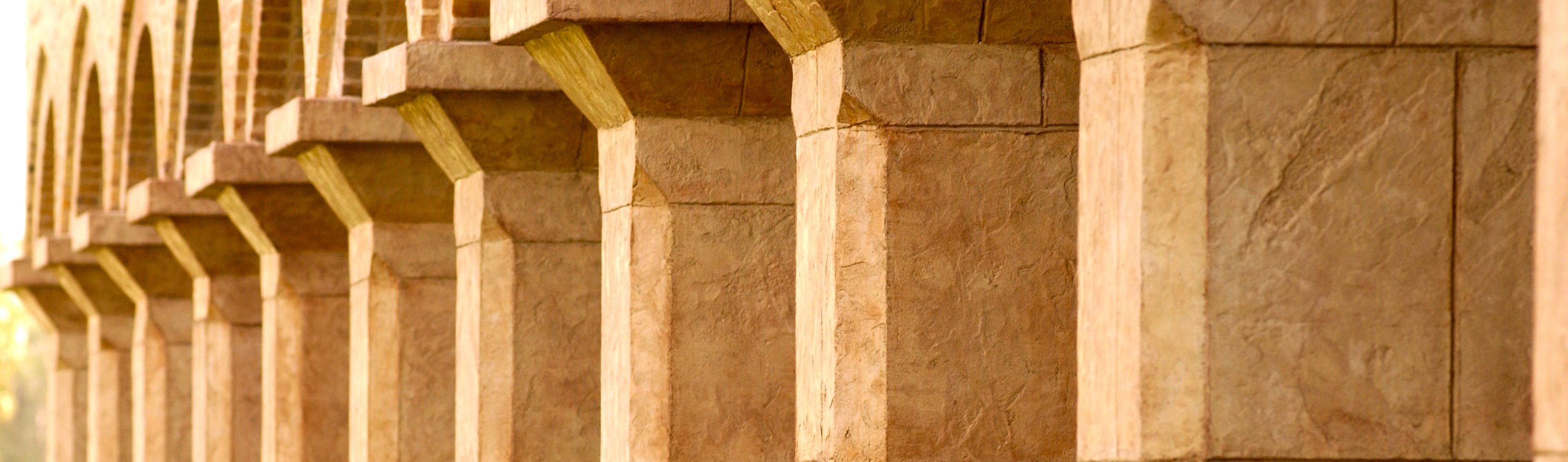
Manufacturing & Industrial Engineering Faculty Publications and Presentations
Document Type
Article
Publication Date
9-2022
Abstract
Three-dimensional scanning is widely used for the dimension measurements of physical objects with freeform designs. The output point cloud is flexible enough to provide a detailed geometric description for these objects. However, geometric accuracy and precision are still debatable for this scanning process. Uncertainties are ubiquitous in geometric measurement due to many physical factors. One potential factor is the object’s posture in the scanning region. The posture of target positioning on the scanning platform could influence the normal of the scanning points, which could further affect the measurement variances. This paper first investigates the geometric and spatial factors that could potentially influence scanning variance. This functional relationship is modeled as a Bayesian extreme learning model, which is later utilized to find the object’s optimal posture for variance reduction. A Bayesian optimization approach is proposed to solve this minimization problem. Case studies are presented to validate the proposed methodology.
Recommended Citation
Geng, Zhaohui, and Bopaya Bidanda. "Automated Posture Positioning for High Precision 3D Scanning of a Freeform Design using Bayesian Optimization." Manufacturing Letters 33 (2022): 802-807. https://doi.org/10.1016/j.mfglet.2022.07.099
Creative Commons License
This work is licensed under a Creative Commons Attribution-NonCommercial-No Derivative Works 4.0 International License.
Publication Title
Manufacturing Letters
DOI
10.1016/j.mfglet.2022.07.099