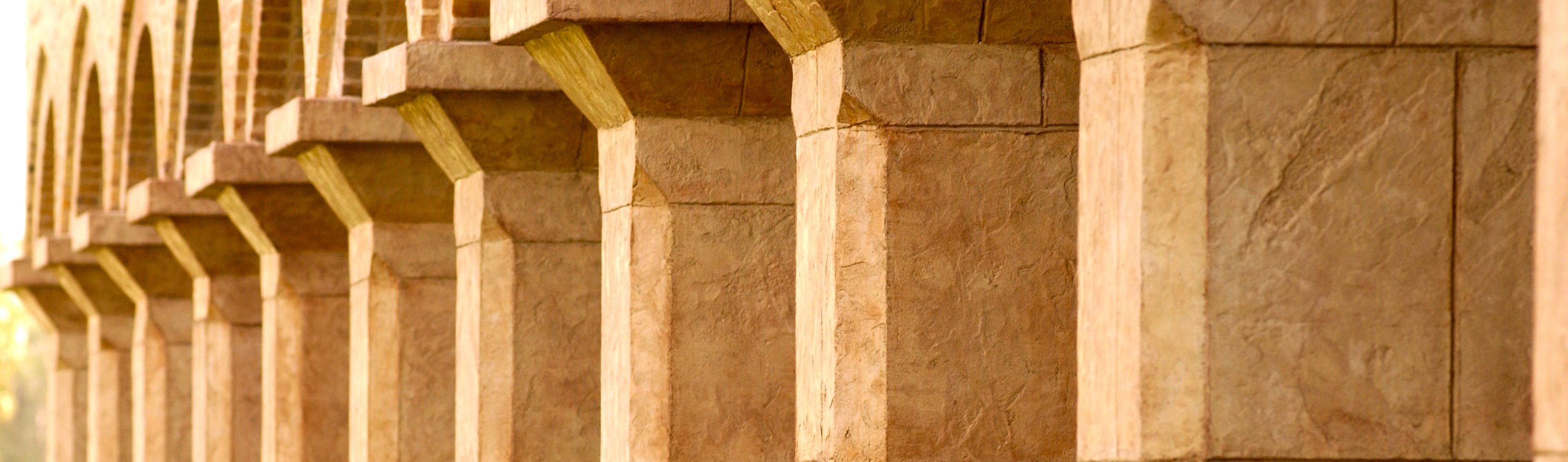
Manufacturing & Industrial Engineering Faculty Publications and Presentations
Automated variance modeling for three-dimensional point cloud data via Bayesian neural networks
Document Type
Article
Publication Date
9-2022
Abstract
Three-dimensional (3-D) point cloud data are increasingly being used to describe a wide range of physical objects in detail, corresponding to customized and flexible shape designs. The advent of a new generation of optical sensors has simplified and reduced the costs of acquiring 3-D data in near-real-time. However, the variation of the acquired point clouds, and methods to describe them, create bottlenecks in manufacturing practices such as Reverse Engineering (RE) and metrology in additive manufacturing. We address this issue by developing an automated variance modeling algorithm that utilizes a physical object’s local geometric descriptors and Bayesian Extreme Learning Machines (BELMs). Our proposed ensemble and residual BELM-variants are trained by a scanning history that is composed of multiple scans of other, distinct objects. The specific scanning history is selected by a new empirical Kullback–Leibler divergence we developed to identify objects that are geometrically similar to an object of interest. A case study of our algorithm on additively manufactured products demonstrates its capability to model the variance of point cloud data for arbitrary freeform shapes based on a scanning history involving simpler, and distinct, shapes. Our algorithm has utility for measuring the process capability of 3-D scanning for RE processes.
Recommended Citation
Zhaohui Geng, Arman Sabbaghi & Bopaya Bidanda (2022) Automated variance modeling for three-dimensional point cloud data via Bayesian neural networks, IISE Transactions, DOI: 10.1080/24725854.2022.2106389
Publication Title
IISE Transactions
DOI
10.1080/24725854.2022.2106389
Comments
Copyright © 2022 “IISE”
https://www.tandfonline.com/share/92HWPDPBCPNYHYHZTPTZ?target=10.1080/24725854.2022.2106389