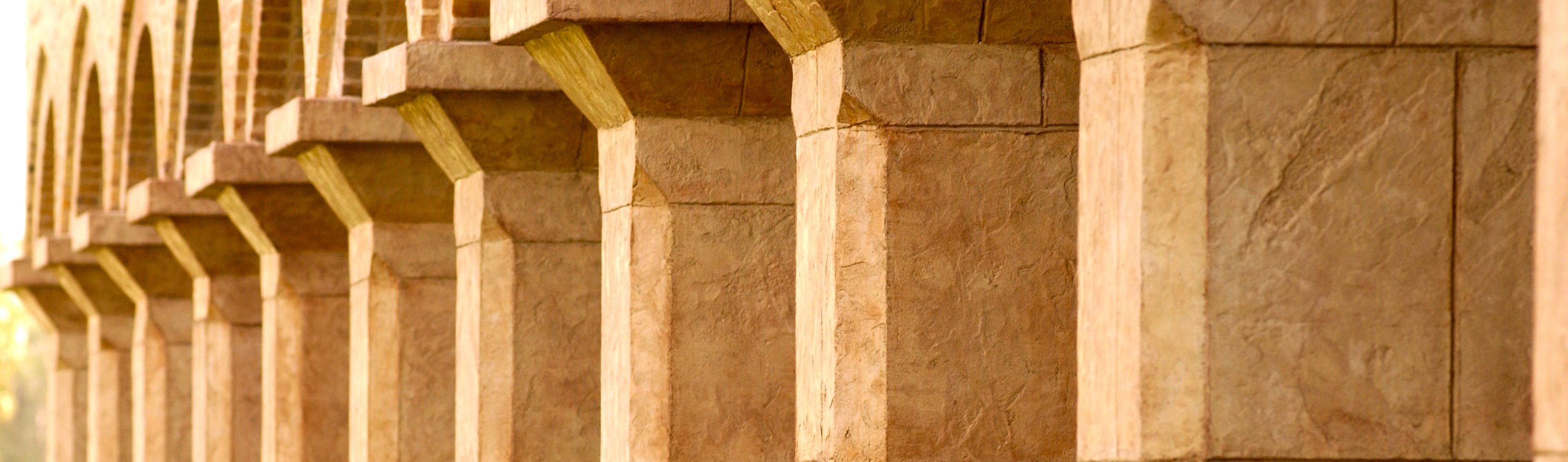
Manufacturing & Industrial Engineering Faculty Publications and Presentations
Document Type
Article
Publication Date
10-2024
Abstract
Achieving control of Laser Powder Bed Fusion (L-PBF) over the quality of the print is the main motivation for finding an optimum set of parameters in the process. Surface roughness is one of the characteristics of the print that impacts the performance of the desired functionality. This research focus is to relate the build angle with the surface roughness on the L-PBF printed specimens and utilize machine learning methods for roughness estimation of geometric features with varying build angles. The EOS M290 L-PBF printer was used to print Inconel-718 coupons using standard process parameters while varying build angles from 20 to 90 degrees at fixed 5-degree intervals. The specimens’ surface was analyzed using metrology tools and the data obtained was used for training the machine learning models. Machine learning methods are used to create regression models for estimating the roughness of the specimens using the build angle and location of the sample on the substrate. The findings of this study provide build angle-based predictive estimation of surface roughness of printed L-PBF parts. The machine learning model will help to make reliable decisions on choosing the build angle of a complex part based on the desired surface roughness of its geometric features.
Recommended Citation
Galarza, Jose, Jorge Barron Jr, Farid Ahmed, and Jianzhi Li. "Data-driven roughness estimation of additively manufactured samples using build angles." Manufacturing Letters 41 (2024): 1092-1099. https://doi.org/10.1016/j.mfglet.2024.09.134
Creative Commons License
This work is licensed under a Creative Commons Attribution-NonCommercial-No Derivative Works 4.0 International License.
Publication Title
Manufacturing Letters
DOI
https://doi.org/10.1016/j.mfglet.2024.09.134
Comments
© 2024 The Author(s). This is an open access article under the CC BY-NC-ND license (https://creativecommons.org/licenses/by-nc-nd/4.0)