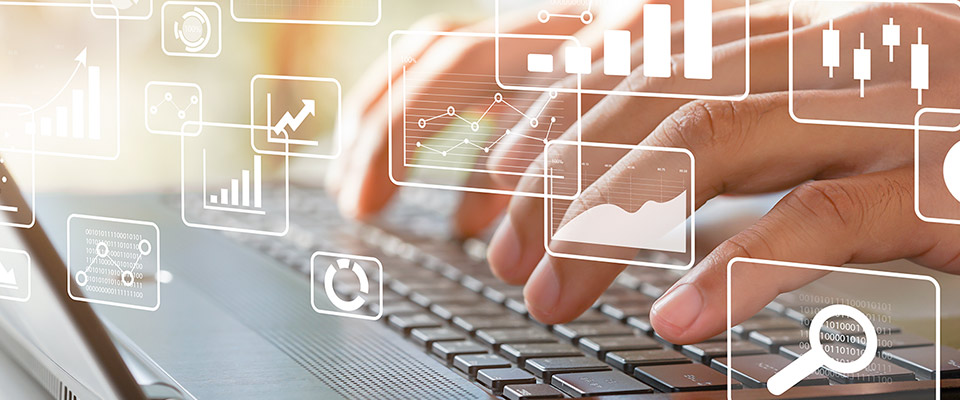
School of Mathematical and Statistical Sciences Faculty Publications and Presentations
Document Type
Article
Publication Date
8-4-2022
Abstract
Background
Hospital length of stay (LOS) is a key indicator of hospital care management efficiency, cost of care, and hospital planning. Hospital LOS is often used as a measure of a post-medical procedure outcome, as a guide to the benefit of a treatment of interest, or as an important risk factor for adverse events. Therefore, understanding hospital LOS variability is always an important healthcare focus. Hospital LOS data can be treated as count data, with discrete and non-negative values, typically right skewed, and often exhibiting excessive zeros. In this study, we compared the performance of the Poisson, negative binomial (NB), zero-inflated Poisson (ZIP), and zero-inflated negative binomial (ZINB) regression models using simulated and empirical data.
Methods
Data were generated under different simulation scenarios with varying sample sizes, proportions of zeros, and levels of overdispersion. Analysis of hospital LOS was conducted using empirical data from the Medical Information Mart for Intensive Care database.
Results
Results showed that Poisson and ZIP models performed poorly in overdispersed data. ZIP outperformed the rest of the regression models when the overdispersion is due to zero-inflation only. NB and ZINB regression models faced substantial convergence issues when incorrectly used to model equidispersed data. NB model provided the best fit in overdispersed data and outperformed the ZINB model in many simulation scenarios with combinations of zero-inflation and overdispersion, regardless of the sample size. In the empirical data analysis, we demonstrated that fitting incorrect models to overdispersed data leaded to incorrect regression coefficients estimates and overstated significance of some of the predictors.
Conclusions
Based on this study, we recommend to the researchers that they consider the ZIP models for count data with zero-inflation only and NB models for overdispersed data or data with combinations of zero-inflation and overdispersion. If the researcher believes there are two different data generating mechanisms producing zeros, then the ZINB regression model may provide greater flexibility when modeling the zero-inflation and overdispersion.
Recommended Citation
Fernandez, G.A., Vatcheva, K.P. A comparison of statistical methods for modeling count data with an application to hospital length of stay. BMC Med Res Methodol 22, 211 (2022). https://doi.org/10.1186/s12874-022-01685-8
Creative Commons License
This work is licensed under a Creative Commons Attribution 4.0 International License.
Publication Title
BMC Medical Research Methodology
DOI
10.1186/s12874-022-01685-8
Comments
© 2022, The Author(s)