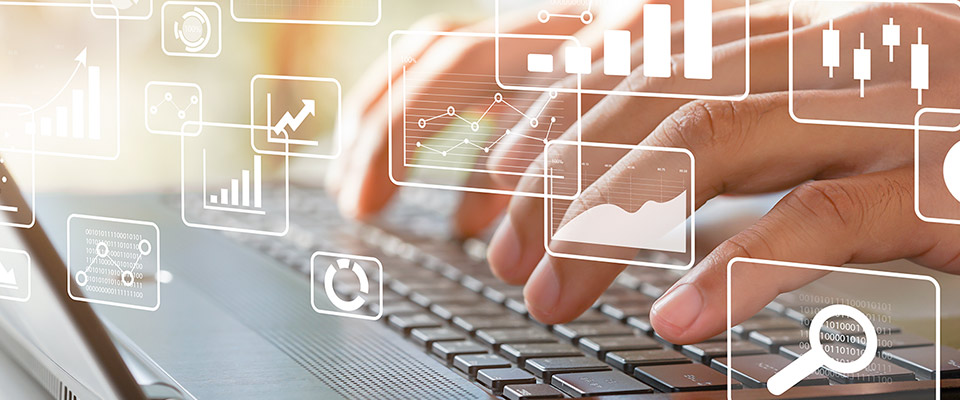
School of Mathematical and Statistical Sciences Faculty Publications and Presentations
Document Type
Article
Publication Date
10-2017
Abstract
Vulnerability forecasting models help us to predict the number of vulnerabilities that may occur in the future for a given Operating System (OS). There exist few models that focus on quantifying future vulnerabilities without consideration of trend, level, seasonality and non linear components of vulnerabilities. Unlike traditional ones, we propose a vulnerability analytic prediction model based on linear and non-linear approaches via time series analysis. We have developed the models based on Auto Regressive Moving Average (ARIMA), Artificial Neural Network (ANN), and Support Vector Machine (SVM) settings. The best model which provides the minimum error rate is selected for prediction of future vulnerabilities. Utilizing time series approach, this study has developed a predictive analytic model for three popular Desktop Operating Systems, namely, Windows 7, Mac OS X, and Linux Kernel by using their reported vulnerabilities on the National Vulnerability Database (NVD). Based on these reported vulnerabilities, we predict ahead their behavior so that the OS companies can make strategic and operational decisions like secure deployment of OS, facilitate backup provisioning, disaster recovery, diversity planning, maintenance scheduling, etc. Similarly, it also helps in assessing current security risks along with estimation of resources needed for handling potential security breaches and to foresee the future releases of security patches. The proposed non-linear analytic models produce very good prediction results in comparison to linear time series models.
Recommended Citation
Pokhrel, N. , Rodrigo, H. and Tsokos, C. (2017) Cybersecurity: Time Series Predictive Modeling of Vulnerabilities of Desktop Operating System Using Linear and Non-Linear Approach. Journal of Information Security, 8, 362-382. https://doi.org/10.4236/jis.2017.84023
Creative Commons License
This work is licensed under a Creative Commons Attribution 4.0 International License.
Publication Title
Journal of Information Security
DOI
10.4236/jis.2017.84023
Comments
Copyright © 2017 by authors and Scientific Research Publishing Inc. This work and the related PDF file are licensed under a Creative Commons Attribution-NonCommercial 4.0 International License.