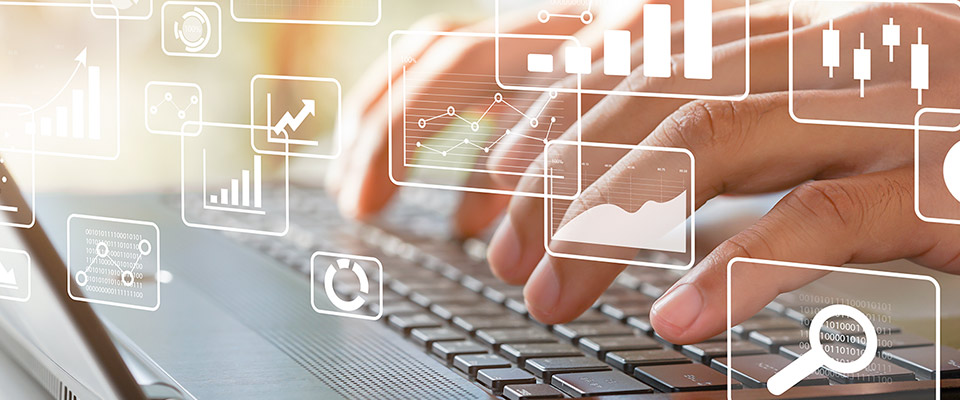
School of Mathematical and Statistical Sciences Faculty Publications and Presentations
Document Type
Article
Publication Date
10-10-2023
Abstract
Background
INTEROCC is a seven-country cohort study of occupational exposures and brain cancer risk, including occupational exposure to electromagnetic fields (EMF). In the absence of data on individual exposures, a Job Exposure Matrix (JEM) may be used to construct likely exposure scenarios in occupational settings. This tool was constructed using statistical summaries of exposure to EMF for various occupational categories for a comparable group of workers.
Methods
In this study, we use the Canadian data from INTEROCC to determine the best EMF exposure surrogate/estimate from three appropriately chosen surrogates from the JEM, along with a fourth surrogate based on Berkson error adjustments obtained via numerical approximation of the likelihood function. In this article, we examine the case in which exposures are gamma-distributed for each occupation in the JEM, as an alternative to the log-normal exposure distribution considered in a previous study conducted by our research team. We also study using those surrogates and the Berkson error adjustment in Poisson regression and conditional logistic regression.
Results
Simulations show that the introduced methods of Berkson error adjustment for non-stratified analyses provide accurate estimates of the risk of developing tumors in case of gamma exposure model. Alternatively, and under some technical assumptions, the arithmetic mean is the best surrogate when a gamma-distribution is used as an exposure model. Simulations also show that none of the present methods could provide an accurate estimate of the risk in case of stratified analyses.
Conclusion
While our previous study found the geometric mean to be the best exposure surrogate, the present study suggests that the best surrogate is dependent on the exposure model; the arithmetic means in case of gamma-exposure model and the geometric means in case of log-normal exposure model. However, we could present a better method of Berkson error adjustment for each of the two exposure models. Our results provide useful guidance on the application of JEMs for occupational exposure assessments, with adjustment for Berkson error.
Recommended Citation
Oraby, T., Chakraborty, S., Sivaganesan, S. et al. Adjusting for Berkson error in exposure in ordinary and conditional logistic regression and in Poisson regression. BMC Med Res Methodol 23, 225 (2023). https://doi.org/10.1186/s12874-023-02044-x
Creative Commons License
This work is licensed under a Creative Commons Attribution 4.0 International License.
Publication Title
BMC Medical Research Methodology
DOI
10.1186/s12874-023-02044-x
Comments
Copyright © 2023, The Author(s)
This is an open access article distributed under the terms of the Creative Commons CC BY license, which permits unrestricted use, distribution, and reproduction in any medium, provided the original work is properly cited.