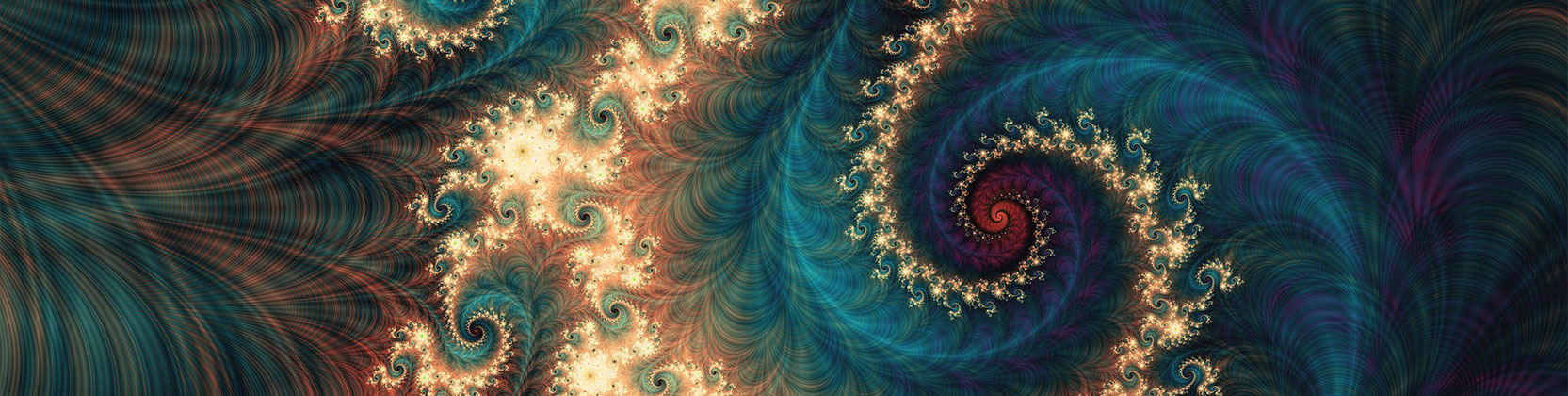
School of Mathematical and Statistical Sciences Faculty Publications and Presentations
Document Type
Article
Publication Date
2-2024
Abstract
In this paper, we propose a sparse Bayesian procedure with global and local(GL) shrinkage priors for the problems of variable selection and classification in high-dimensional logistic regression models. In particular, we consider two types of GL shrinkage priors for the regression coefficients, the horseshoe (HS)prior and the normal-gamma (NG) prior, and then specify a correlated prior for the binary vector to distinguish models with the same size. The GL priors are then combined with mixture representations of logistic distribution to construct a hierarchical Bayes model that allows efficient implementation of a Markov chain Monte Carlo (MCMC) to generate samples from posterior distribution. We carry out simulations to compare the finite sample performances of the proposed Bayesian method with the existing Bayesian methods in terms of the accuracy of variable selection and prediction. Finally, two real-data applications are provided for illustrative purposes.
Recommended Citation
Ma, Zhuanzhuan, Zifei Han, Souparno Ghosh, Liucang Wu, and Min Wang. "Sparse Bayesian variable selection in high‐dimensional logistic regression models with correlated priors." Statistical Analysis and Data Mining: The ASA Data Science Journal 17, no. 1 (2024): e11663. https://doi.org/10.1002/sam.11663
Publication Title
Statistical Analysis and Data Mining: The ASA Data Science Journal
DOI
10.1002/sam.11663
Included in
Applied Statistics Commons, Data Science Commons, Statistical Methodology Commons, Statistical Models Commons
Comments
Original published version available at https://doi.org/10.1002/sam.11663
https://onlinelibrary.wiley.com/share/VKGYY2YVDH5RCTWVKPTW?target=10.1002/sam.11663