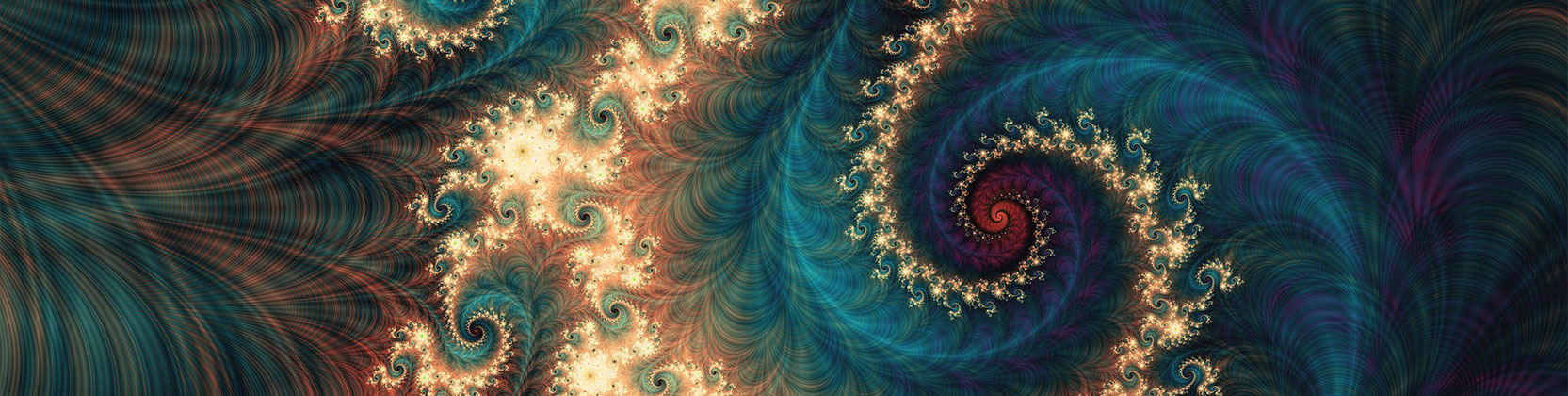
School of Mathematical and Statistical Sciences Faculty Publications and Presentations
Document Type
Article
Publication Date
3-15-2024
Abstract
In this paper, we explore a new idea of using deep learning representations as a principle for regularization in inverse problems for digital signal processing. Specifically, we consider the standard variational formulation, where a composite function encodes a fidelity term that quantifies the proximity of the candidate solution to the observations (under a physical process), and a second regularization term that constrains the space of solutions according to some prior knowledge. In this work, we investigate deep learning representations as a means of fulfilling the role of this second (regularization) term. Several numerical examples are presented for signal restoration under different degradation processes, showing successful recovery under the proposed methodology. Moreover, one of these examples uses real data on energy usage by households in London from 2012 to 2014.
Recommended Citation
Ramirez Villamarin, Carlos, Erwin Suazo, and Tamer Oraby. "Regularization by deep learning in signal processing." Signal, Image and Video Processing 18, no. 5 (2024): 4425-4433. https://doi.org/10.1007/s11760-024-03083-7
Publication Title
Signal, Image and Video Processing
DOI
10.1007/s11760-024-03083-7
Comments
This version of the article has been accepted for publication, after peer review (when applicable) and is subject to Springer Nature’s AM terms of use, but is not the Version of Record and does not reflect post-acceptance improvements, or any corrections. The Version of Record is available online at: https://doi.org/10.1007/s11760-024-03083-7
https://rdcu.be/dEOut