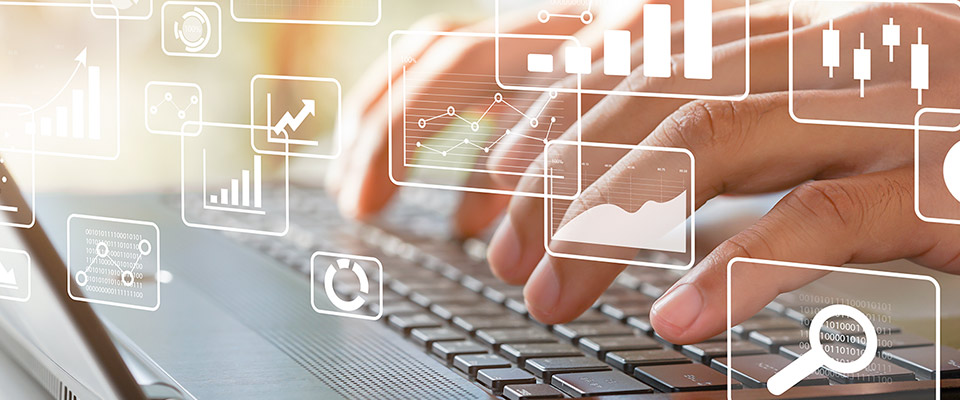
School of Mathematical and Statistical Sciences Faculty Publications and Presentations
Document Type
Article
Publication Date
11-30-2020
Abstract
We present an unsupervised method to detect anomalous time series among a collection of time series. To do so, we extend traditional Kernel Density Estimation for estimating probability distributions in Euclidean space to Hilbert spaces. The estimated probability densities we derive can be obtained formally through treating each series as a point in a Hilbert space, placing a kernel at those points, and summing the kernels (a “point approach”), or through using Kernel Density Estimation to approximate the distributions of Fourier mode coefficients to infer a probability density (a “Fourier approach”). We refer to these approaches as Functional Kernel Density Estimation for Anomaly Detection as they both yield functionals that can score a time series for how anomalous it is. Both methods naturally handle missing data and apply to a variety of settings, performing well when compared with an outlyingness score derived from a boxplot method for functional data, with a Principal Component Analysis approach for functional data, and with the Functional Isolation Forest method. We illustrate the use of the proposed methods with aviation safety report data from the International Air Transport Association (IATA).
Recommended Citation
Lindstrom, Michael R., Hyuntae Jung, and Denis Larocque. "Functional Kernel Density Estimation: Point and Fourier Approaches to Time Series Anomaly Detection." Entropy 22, no. 12 (2020): 1363. https://doi.org/10.3390%2Fe22121363
Creative Commons License
This work is licensed under a Creative Commons Attribution 4.0 International License.
Publication Title
Entropy
DOI
10.3390/e22121363
Comments
Copyright © 2020 by the authors. Licensee MDPI, Basel, Switzerland. This article is an open access article distributed under the terms and conditions of the Creative Commons Attribution (CC BY) license (http://creativecommons.org/licenses/by/4.0/).