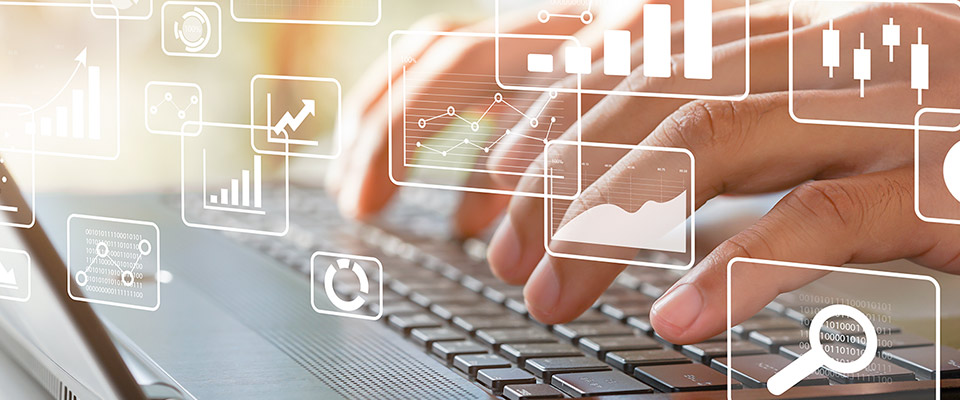
School of Mathematical and Statistical Sciences Faculty Publications and Presentations
Document Type
Article
Publication Date
12-2024
Abstract
This study presents a comprehensive evaluation of five prominent unsupervised machine learning anomaly detection algorithms: One-Class Support Vector Machine (One-Class SVM), One-Class SVM with Stochastic Gradient Descent (SGD), Isolation Forest (iForest), Local Outlier Factor (LOF), and Robust Covariance (Elliptic Envelope). Through systematic analysis on a synthetically simulated dataset, the study assessed each algorithm’s predictive performance using accuracy, precision, recall, and F1 score specifically for outlier detection. The evaluation reveals that One-Class SVM, Isolation Forest, and Robust Covariance are more effective in identifying outliers in the synthetic simulated dataset, with Isolation Forest slightly outperforming the other algorithms in terms of balancing precision and recall. One-Class SVM with SGD shows promise in precision but needs adjustment to improve recall. Local Outlier Factor may require parameter tuning or may not be as suitable for this particular dataset’s characteristics. The findings reveal significant variations in performance, highlighting the strengths and limitations of each method in identifying anomalies. This research contributes to the field of machine learning by demonstrating that the selection of an anomaly detection algorithm should be a considered decision, taking into account the specific characteristics of the data and the operational context of its application. Future work should explore parameter optimization, the impact of dataset characteristics on model performance, and the application of these models to real-world datasets to validate their efficacy in practical anomaly detection scenarios.
Recommended Citation
Agyemang, Edmund Fosu. "Anomaly detection using unsupervised machine learning algorithms: A simulation study." Scientific African 26 (2024): e02386. https://doi.org/10.1016/j.sciaf.2024.e02386
Creative Commons License
This work is licensed under a Creative Commons Attribution 4.0 International License.
Publication Title
Scientific African
DOI
10.1016/j.sciaf.2024.e02386
Comments
Under a Creative Commons license http://creativecommons.org/licenses/by/4.0/