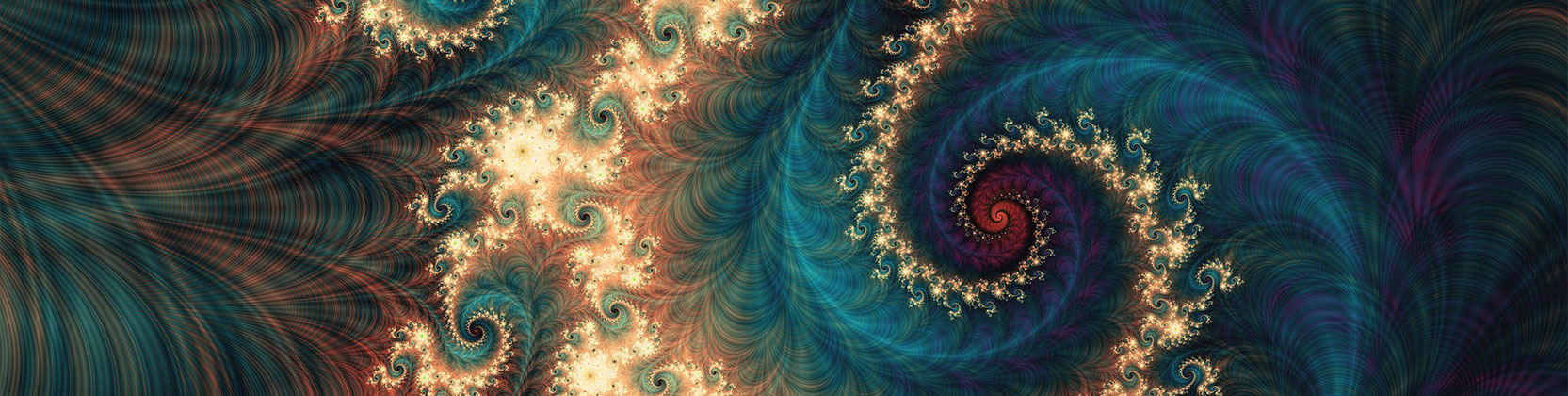
School of Mathematical and Statistical Sciences Faculty Publications and Presentations
Document Type
Article
Publication Date
12-13-2024
Abstract
Background
Snakebite envenoming is a serious condition that affects 2.5 million people and causes 81,000–138,000 deaths every year, particularly in tropical and subtropical regions. The World Health Organization has set a goal to halve the deaths and disabilities related to snakebite envenoming by 2030. However, significant challenges in achieving this goal include a lack of robust research evidence related to snakebite incidence and treatment, particularly in sub-Saharan Africa. This study aimed to combine established methodologies with the latest tools in Artificial Intelligence to assess the barriers to effective snakebite treatment in Ghana.
Method
We used a MaxDiff statistical experiment design to collect data, and six supervised machine learning models were applied to predict responses whose performance showed an advantage over the other through 6921 data points partitioned using the hold-back validation method, with 70% training and 30% validation. The results were compared using key metrics: Akaike Information Criterion corrected, Bayesian Information Criterion, Root Average Squared Error, and Fit Time in milliseconds.
Results
Considering all the responses, none of the six machine learning algorithms proved superior, but the Generalized Regression Model (Ridge) performed consistently better among the candidate models. The model consistently predicted several key significant barriers to effective snakebite treatment, such as the high cost of antivenoms, increased use of unorthodox, harmful practices, lack of access to effective antivenoms in remote areas when needed, and resorting to unorthodox and harmful practices in addition to hospital treatment.
Conclusion
The combination of a MaxDiff statistical experiment design to collect data and six machine learning models allowed the identification of barriers to accessing effective therapies for snakebite envenoming in Ghana. Addressing these barriers through targeted policy interventions, including intensified advocacy, continuous education, community engagement, healthcare worker training, and strategic investments, can enhance the effectiveness of snakebite treatment, ultimately benefiting snakebite victims and reducing the burden of snakebite envenoming. There is a need for robust regulatory frameworks and increased antivenom production to address these barriers.
Author summary
Snakebite envenoming, a severe condition, affects 2.5 million people and causes 81,000–138,000 deaths annually, particularly in tropical and subtropical regions of Africa, Asia, and Latin America. The World Health Organization aims to reduce this burden by 50% by 2030. However, significant barriers exist to achieving these targets, especially in Sub-Saharan Africa. These barriers include limited rigorous research evidence and the lack of investment in effective antivenoms for local snake species. Meeting this goal will require innovative research to gather better data on snakebite incidence and treatment. Artificial intelligence is one promising field that can contribute to this effort. For the first time, we have demonstrated how MaxDiff statistical experiment designs and machine learning algorithms can be explored to predict the barriers to effective snakebite treatment, such as the high cost of antivenoms, increased use of harmful practices, lack of access to effective antivenoms in remote areas, and resorting to unorthodox and harmful practices in addition to hospital treatment. Addressing these barriers through targeted policy interventions, including intensified advocacy, continuous education, community engagement, healthcare worker training, and strategic investments, can enhance the effectiveness of snakebite treatment. Robust regulatory frameworks and increased local antivenom production are also needed to address these barriers.
Recommended Citation
Nyarko, Eric, Edmund Fosu Agyemang, Ebenezer Kwesi Ameho, Louis Agyekum, José María Gutiérrez, and Eduardo Alberto Fernandez. "A supervised machine learning statistical design of experiment approach to modeling the barriers to effective snakebite treatment in Ghana." PLOS Neglected Tropical Diseases 18, no. 12 (2024): e0012736. https://doi.org/10.1371/journal.pntd.0012736
Creative Commons License
This work is licensed under a Creative Commons Attribution 4.0 International License.
Publication Title
PLOS Neglected Tropical Diseases
DOI
10.1371/journal.pntd.0012736
Comments
Student publication.
© 2024 Nyarko et al. This is an open access article distributed under the terms of the Creative Commons Attribution License, which permits unrestricted use, distribution, and reproduction in any medium, provided the original author and source are credited.