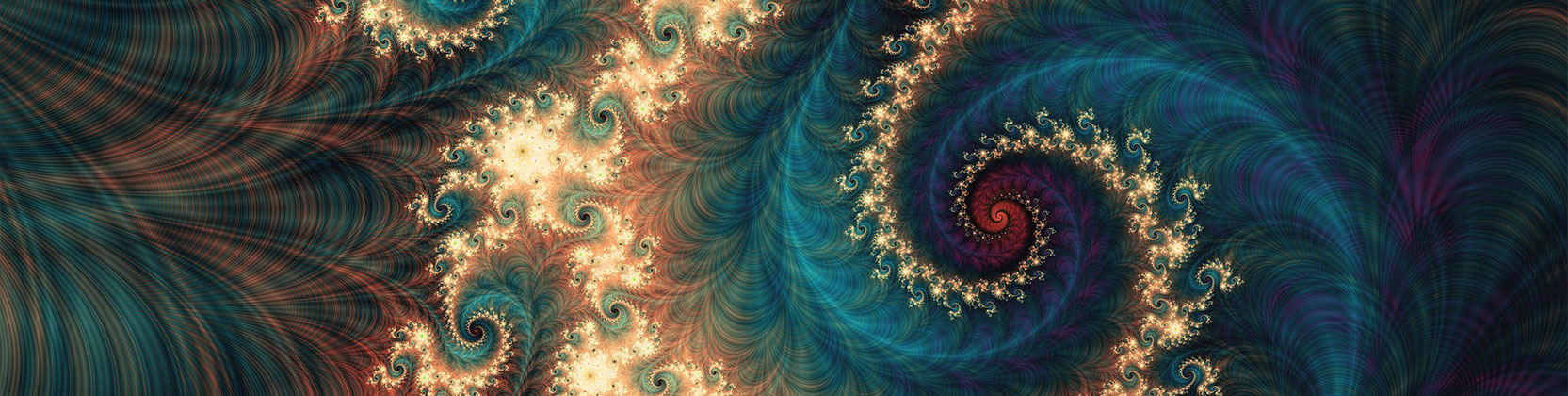
School of Mathematical and Statistical Sciences Faculty Publications and Presentations
Bayesian Nonparametric Hypothesis Testing Methods on Multiple Comparisons
Document Type
Article
Publication Date
3-12-2025
Abstract
In this paper, we introduce Bayesian testing procedures based on the Bayes factor to compare the means across multiple populations in classical nonparametric contexts. The proposed Bayesian methods are designed to maximize the probability of rejecting the null hypothesis when the Bayes factor exceeds a specified evidence threshold. It is shown that these procedures have straightforward closed-form expressions based on classical nonparametric test statistics and their corresponding critical values, allowing for easy computation. We also demonstrate that they effectively control Type I error and enable researchers to make consistent decisions aligned with both frequentist and Bayesian approaches, provided that the evidence threshold for the Bayesian methods is set according to the significance level of the frequentist tests. Importantly, the proposed approaches allow for the quantification of evidence from empirical data in favor of the null hypothesis, an advantage that frequentist methods lack, as they cannot quantify support for the null when the null hypothesis is not rejected. We also present simulation studies and real-world applications to illustrate the performance of the proposed testing procedures.
Recommended Citation
Hai, Qiuchen, and Zhuanzhuan Ma. 2025. “Bayesian Nonparametric Hypothesis Testing Methods on Multiple Comparisons.” Computational Statistics, March. https://doi.org/10.1007/s00180-025-01615-4
Publication Title
Computational Statistics
DOI
10.1007/s00180-025-01615-4
Comments
Reprints and permissions