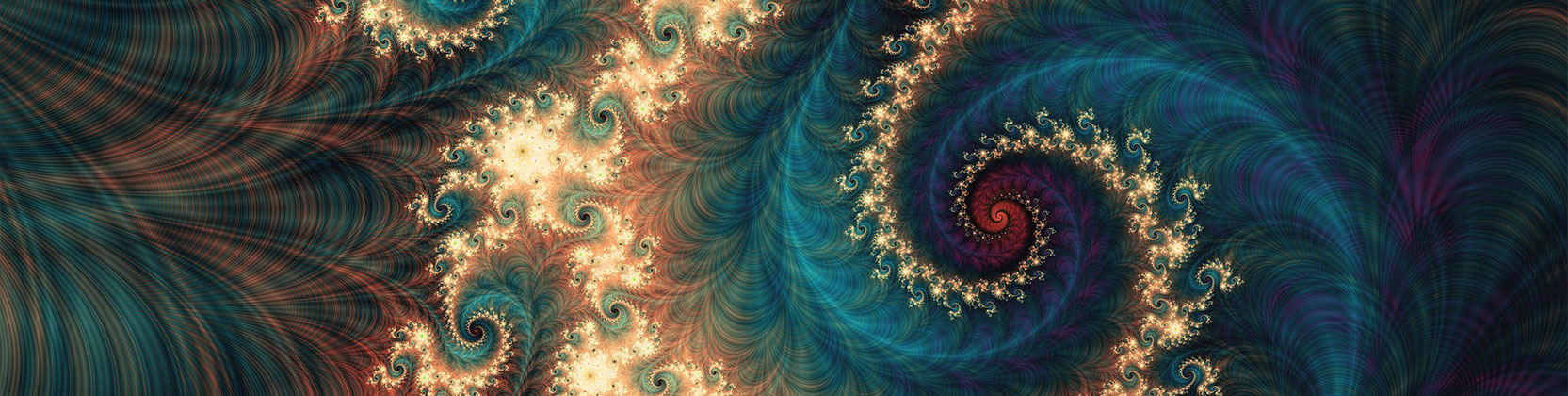
School of Mathematical and Statistical Sciences Faculty Publications and Presentations
Document Type
Article
Publication Date
11-27-2017
Abstract
In this study, a hybrid method based on coupling discrete wavelet transforms (DWTs) and artificial neural network (ANN) for yield spread forecasting is proposed. The discrete wavelet transform (DWT) using five different wavelet families is applied to decompose the five different yield spreads constructed at shorter end, longer end, and policy relevant area of the yield curve to eliminate noise from them. The wavelet coefficients are then used as inputs into Levenberg-Marquardt (LM) ANN models to forecast the predictive power of each of these spreads for output growth. We find that the yield spreads constructed at the shorter end and policy relevant areas of the yield curve have a better predictive power to forecast the output growth, whereas the yield spreads, which are constructed at the longer end of the yield curve do not seem to have predictive information for output growth. These results provide the robustness to the earlier results.
Recommended Citation
Shah, Firdous Ahmad, and Lokenath Debnath. 2017. "Wavelet Neural Network Model for Yield Spread Forecasting" Mathematics 5, no. 4: 72. https://doi.org/10.3390/math5040072
Creative Commons License
This work is licensed under a Creative Commons Attribution 4.0 International License.
Publication Title
Mathematics
DOI
10.3390/math5040072
Comments
© 2017 by the authors. Licensee MDPI, Basel, Switzerland. This article is an open access article distributed under the terms and conditions of the Creative Commons Attribution (CC BY) license (http://creativecommons.org/licenses/by/4.0/).