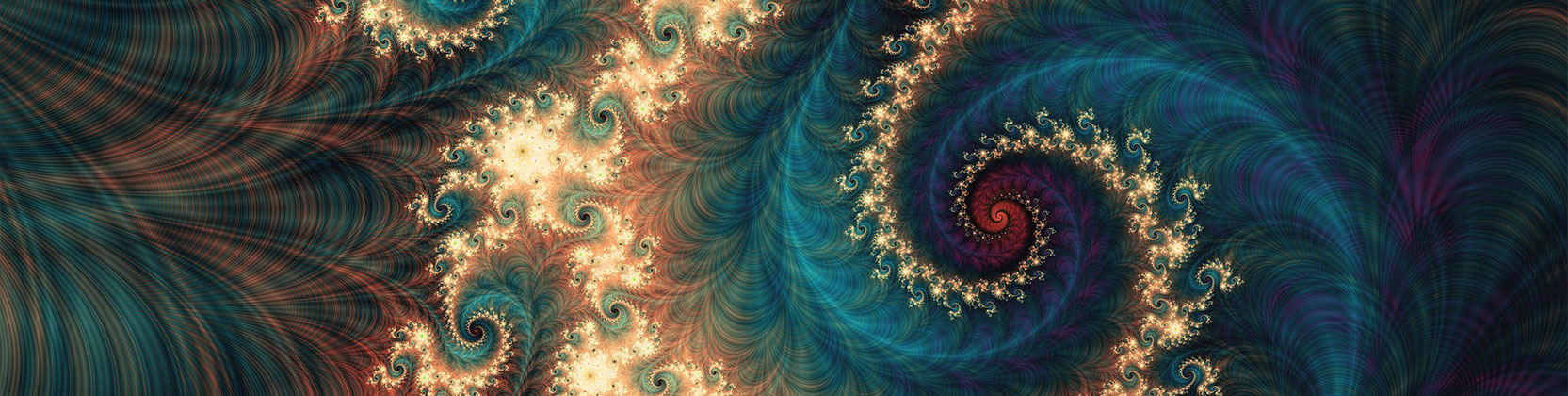
School of Mathematical and Statistical Sciences Faculty Publications and Presentations
Document Type
Article
Publication Date
2-26-2025
Abstract
While analyzing health data is important for improving health outcomes, class imbalance in datasets poses major challenges to machine learning classification models. This work, therefore, considers the class imbalance problem in stroke prediction using models such as K-nearest neighbors, support vector machine, logistic regression, random forest, and decision tree. This work balances the stroke dataset, thereby enhancing model performance, through various oversampling strategies: random oversampling (RO), ADASYN, SMOTE, and SMOTE–Tomek. Compared to the results of the imbalanced dataset, all applied oversampling techniques enhanced the correct classification of stroke events by the ML model. Among these, RO–SVM with RBF kernel was the best in terms of sensitivity, specificity, G-mean, F1-score, and accuracy values, offering the highest results with respective values of 89.87%, 94.91%, 92.36%, 89.64%, and 89.87%. After applying oversampling techniques, all the machine learning classifications were good enough to classify stroke status, especially for the minority class. This study has highlighted the importance of class imbalance issues in health datasets. Precise detection of instances of minority classes can be enhanced considerably by employing classification models with the implementation of hybrid strategies to effectively solve class imbalance issues, which, in turn, will help improve healthcare outcomes. Further research in integrating more advanced deep learning techniques into other health datasets with imbalances is encouraged to further validate or refine class imbalance approaches, as effective handling of imbalanced classes can substantially promote predictive model performance in the analysis of healthcare.
Recommended Citation
Agyemang, Edmund Fosu, Joseph Agyapong Mensah, Eric Nyarko, Dennis Arku, Benedict Mbeah-Baiden, Enock Opoku, and Ezekiel Nii Noye Nortey. "Addressing Class Imbalance Problem in Health Data Classification: Practical Application From an Oversampling Viewpoint." Applied Computational Intelligence and Soft Computing 2025, no. 1 (2025): 1013769. https://doi.org/10.1155/acis/1013769
Creative Commons License
This work is licensed under a Creative Commons Attribution 4.0 International License.
Publication Title
Applied Computational Intelligence and Soft Computing
DOI
10.1155/acis/1013769
Comments
Student publication.
Copyright © 2025 Edmund Fosu Agyemang et al. Applied Computational Intelligence and Soft Computing published by John Wiley & Sons Ltd.
This is an open access article under the terms of the Creative Commons Attribution License, which permits use, distribution and reproduction in any medium, provided the original work is properly cited.