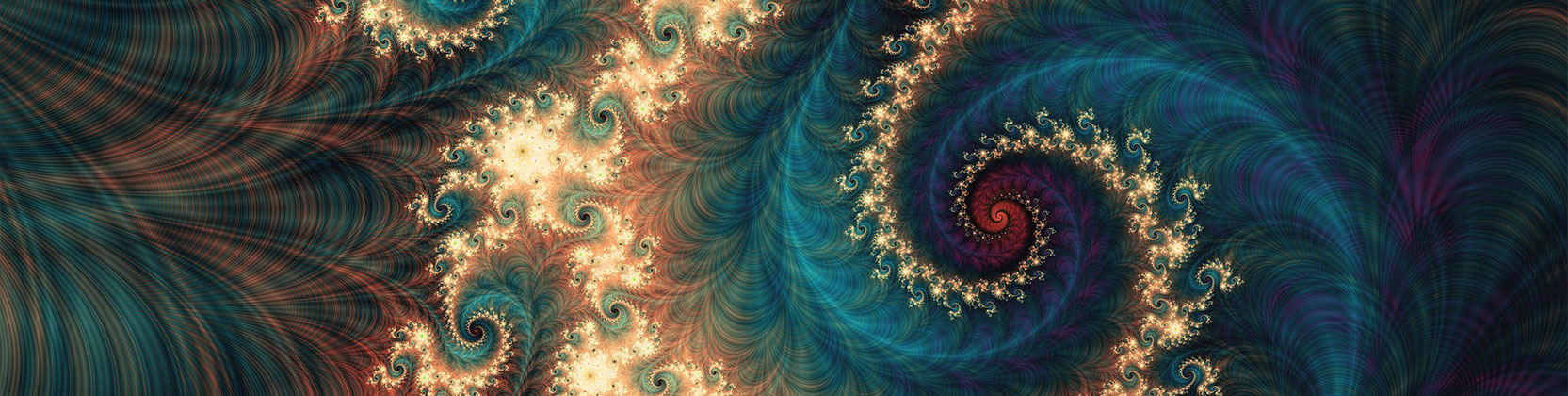
School of Mathematical and Statistical Sciences Faculty Publications and Presentations
Document Type
Article
Publication Date
3-5-2025
Abstract
Heart disease stands as a leading cause of morbidity and mortality globally, presenting a significant public health challenge. Therefore, early prediction and detection are critical, leading to timely and appropriate interventions at early stages. Four ensemble tree-based algorithms were used in this study: adaptive boosting, extreme gradient boosting, random forest, and extremely randomized trees, investigating their ability to predict heart disease. Data related to heart disease clinical features was obtained from the open Kaggle Machine Learning Dataset repository. Adaptive Boosting stands out as the highest performer, achieving an average testing accuracy of 93.70%, precision of 93.71%, recall of 93.70%, and F1 score of 93.69%, along with the highest AUC score of 0.9708, across all competing models considered in the study. These metrics indicate a superior ability to distinguish between patients with and without heart disease, effectively making it particularly valuable for clinical applications where early detection can save lives. The SHapley Additive exPlanations (SHAP) framework adopted to investigate the relative importance of the features in predicting heart disease revealed the most influential predictors (ST slope, chest pain type, old peak, and cholesterol), further aiding the understanding of heart disease mechanisms. Future work should explore the integration of ensemble learning algorithms with real-time patient monitoring systems. This integration could allow for continuous health status updates, equipping predictive models with the information necessary to facilitate dynamic, real-time interventions that are more closely aligned with patient needs.
Recommended Citation
Sakyi-Yeboah, Enoch, Edmund Fosu Agyemang, Vincent Agbenyeavu, Akua Osei-Nkwantabisa, Priscilla Kissi-Appiah, Lateef Moshood, Lawrence Agbota, and Ezekiel NN Nortey. "Heart Disease Prediction Using Ensemble Tree Algorithms: A Supervised Learning Perspective." Applied Computational Intelligence and Soft Computing 2025, no. 1 (2025): 1989813. https://doi.org/10.1155/acis/1989813
DOI
10.1155/acis/1989813
Comments
© 2025 Enoch Sakyi-Yeboah et al. Applied Computational Intelligence and Soft Computing published by John Wiley & Sons Ltd.
This is an open access article under the terms of the Creative Commons Attribution License, which permits use, distribution and reproduction in any medium, provided the original work is properly cited.