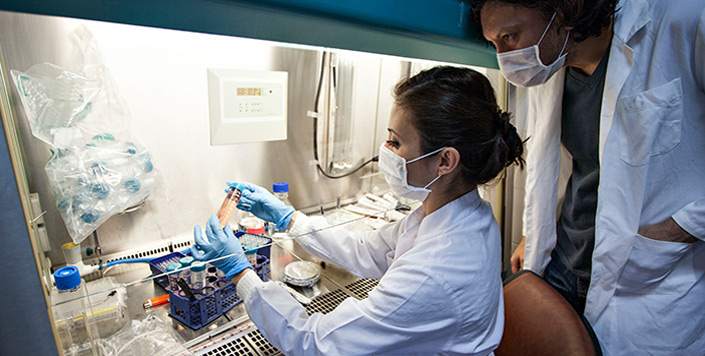
School of Medicine Publications and Presentations
Detecting clinical practice guideline-recommended wheelchair propulsion patterns with wearable devices following a wheelchair propulsion intervention
Document Type
Article
Publication Date
3-2023
Abstract
Wheelchair propulsion interventions typically teach manual wheelchair users to perform wheelchair propulsion biomechanics as recommended by the Clinical Practice Guidelines (CPG). Outcome measures for these interventions are primarily laboratory based. Discrepancies remain between manual wheelchair propulsion (MWP) in laboratory-based examinations and propulsion in the real-world. Current developments in machine learning (ML) allow for monitoring of MWP in the real world. In this study, we collected data from participants enrolled in two wheelchair propulsion interventions, then built an ML algorithm to distinguish CPG recommended MWP patterns from non-CPG-recommended patterns. Eight primary manual wheelchair users did not initially follow CPG recommendations but learned and performed CPG propulsion after the interventions. Participants each wore two inertial measurement units as they propelled their wheelchairs on a roller system, indoors overground, and outdoors. ML models were trained to classify propulsion patterns as following the CPG or not following the CPG. Video recordings were used for reference. For indoor detection, we found that a subject-independent model was able to achieve 85% accuracy. For outdoor detection, we found that the subject-independent model achieved 75.4% accuracy. These results provide further evidence that CPG and non-CPG-recommended MWP patterns can be predicted with wearable sensors using an ML algorithm.
Recommended Citation
Chen, P. W., Klaesner, J., Zwir, I., & Morgan, K. A. (2023). Detecting clinical practice guideline-recommended wheelchair propulsion patterns with wearable devices following a wheelchair propulsion intervention. Assistive Technology, 35(2), 193-201. https://doi.org/10.1080/10400435.2021.2010146
Publication Title
Assistive Technology
DOI
10.1080/10400435.2021.2010146
Academic Level
faculty
Comments
© 2022 RESNA
https://www.tandfonline.com/share/Z4QQNDYPSBNEPIIP236D?target=10.1080/10400435.2021.2010146