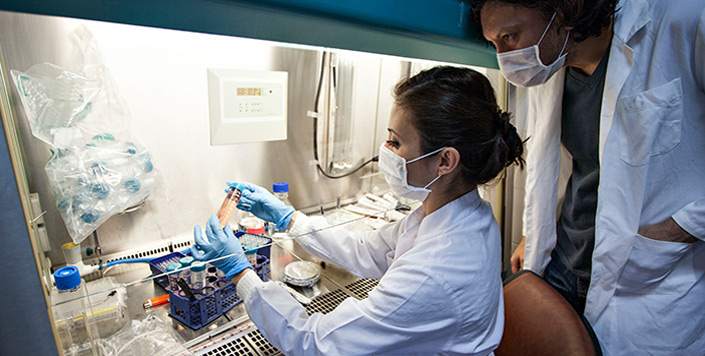
School of Medicine Publications and Presentations
Document Type
Article
Publication Date
4-27-2022
Abstract
Influential theories implicate variations in the mechanisms supporting threat learning in the severity of anxiety symptoms. We use computational models of associative learning in conjunction with structural imaging to explicate links among the mechanisms underlying threat learning, their neuroanatomical substrates, and anxiety severity in humans. We recorded skin-conductance data during a threat-learning task from individuals with and without anxiety disorders (N=251; 8-50 years; 116 females). Reinforcement-learning model variants quantified processes hypothesized to relate to anxiety: threat conditioning, threat generalization, safety learning, and threat extinction. We identified the best-fitting models for these processes and tested associations among latent learning parameters, whole-brain anatomy, and anxiety severity. Results indicate that greater anxiety severity related specifically to slower safety learning and slower extinction of response to safe stimuli. Nucleus accumbens gray-matter volume moderated learning-anxiety associations. Using a modeling approach, we identify computational mechanisms linking threat learning and anxiety severity and their neuroanatomical substrates.
Recommended Citation
Abend, R., Burk, D., Ruiz, S. G., Gold, A. L., Napoli, J. L., Britton, J. C., ... & Averbeck, B. B. (2022). Computational modeling of threat learning reveals links with anxiety and neuroanatomy in humans. eLife, 11, e66169. https://doi.org/10.7554/eLife.66169
Publication Title
eLife
DOI
10.7554/eLife.66169
Academic Level
faculty
Mentor/PI Department
Office of Human Genetics
Comments
This is an open-access article, free of all copyright, and may be freely reproduced, distributed, transmitted, modified, built upon, or otherwise used by anyone for any lawful purpose. The work is made available under the Creative Commons CC0 public domain dedication.