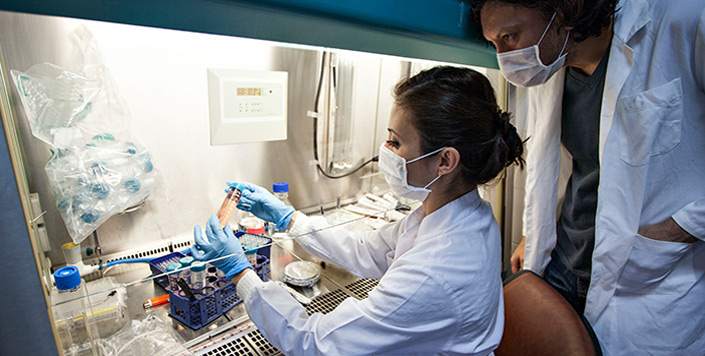
School of Medicine Publications and Presentations
Document Type
Article
Publication Date
8-15-2019
Abstract
There are a wealth of tools for fitting linear models at each location in the brain in neuroimaging analysis, and a wealth of genetic tools for estimating heritability for a small number of phenotypes. But there remains a need for computationally efficient neuroimaging genetic tools that can conduct analyses at the brain-wide scale. Here we present a simple method for heritability estimation on twins that replaces a variance component model-which requires iterative optimisation-with a (noniterative) linear regression model, by transforming data to squared twin-pair differences. We demonstrate that the method has comparable bias, mean squared error, false positive risk, and power to best practice maximum-likelihood-based methods, while requiring a small fraction of the computation time. Combined with permutation, we call this approach "Accelerated Permutation Inference for the ACE Model (APACE)" where ACE refers to the additive genetic (A) effects, and common (C), and unique (E) environmental influences on the trait. We show how the use of spatial statistics like cluster size can dramatically improve power, and illustrate the method on a heritability analysis of an fMRI working memory dataset.
Recommended Citation
Chen, X., Formisano, E., Blokland, G. A., Strike, L. T., McMahon, K. L., de Zubicaray, G. I., ... & Nichols, T. E. (2019). Accelerated estimation and permutation inference for ACE modeling. Human brain mapping, 40(12), 3488-3507. https://doi.org/10.1002/hbm.24611
Creative Commons License
This work is licensed under a Creative Commons Attribution 4.0 International License.
First Page
3488
Last Page
3507
Publication Title
Human brain mapping
DOI
10.1002/hbm.24611
Academic Level
faculty
Mentor/PI Department
Office of Human Genetics
Comments
Copyright © 2019 The Authors.