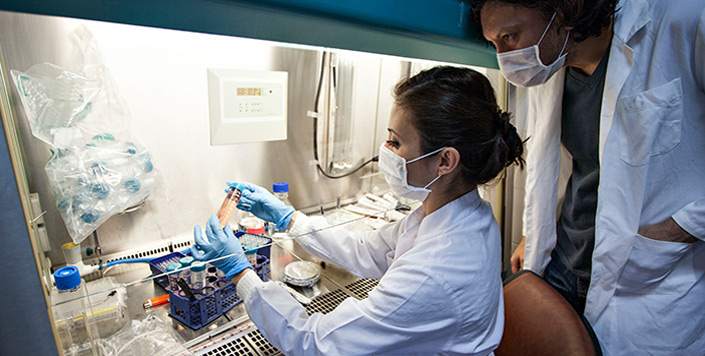
School of Medicine Publications and Presentations
Document Type
Article
Publication Date
9-2017
Abstract
Inferring relatedness from genomic data is an essential component of genetic association studies, population genetics, forensics, and genealogy. While numerous methods exist for inferring relatedness, thorough evaluation of these approaches in real data has been lacking. Here, we report an assessment of 12 state-of-the-art pairwise relatedness inference methods using a data set with 2485 individuals contained in several large pedigrees that span up to six generations. We find that all methods have high accuracy (92–99%) when detecting first- and second-degree relationships, but their accuracy dwindles to76% of relative pairs. Overall, the most accurate methods are Estimation of Recent Shared Ancestry (ERSA) and approaches that compute total IBD sharing using the output from GERMLINE and Refined IBD to infer relatedness. Combining information from the most accurate methods provides little accuracy improvement, indicating that novel approaches, such as new methods that leverage relatedness signals from multiple samples, are needed to achieve a sizeable jump in performance.
Recommended Citation
Ramstetter, Monica D., Thomas D. Dyer, Donna M. Lehman, Joanne E. Curran, Ravindranath Duggirala, John Blangero, Jason G. Mezey, and Amy L. Williams. 2017. “Benchmarking Relatedness Inference Methods with Genome-Wide Data from Thousands of Relatives.” Genetics 207 (1): 75–82. https://doi.org/10.1534/genetics.117.1122.
Creative Commons License
This work is licensed under a Creative Commons Attribution 4.0 International License.
Publication Title
Genetics
DOI
10.1534/genetics.117.1122
Academic Level
faculty
Mentor/PI Department
Office of Human Genetics
Comments
© 2017 Ramstetter et al.