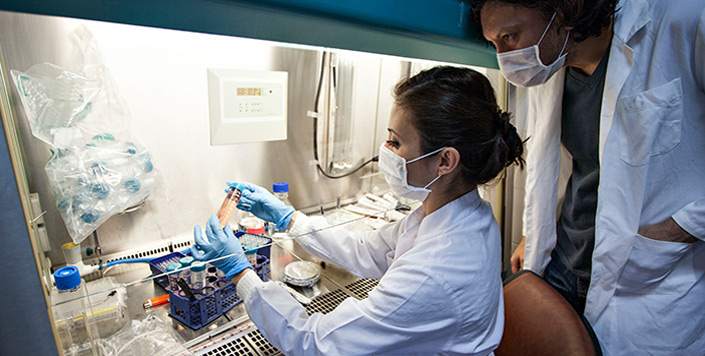
School of Medicine Publications and Presentations
Efficient Variant Set Mixed Model Association Tests for Continuous and Binary Traits in Large-Scale Whole-Genome Sequencing Studies
Document Type
Article
Publication Date
2-7-2019
Abstract
With advances in whole-genome sequencing (WGS) technology, more advanced statistical methods for testing genetic association with rare variants are being developed. Methods in which variants are grouped for analysis are also known as variant-set, gene-based, and aggregate unit tests. The burden test and sequence kernel association test (SKAT) are two widely used variant-set tests, which were originally developed for samples of unrelated individuals and later have been extended to family data with known pedigree structures. However, computationally efficient and powerful variant-set tests are needed to make analyses tractable in large-scale WGS studies with complex study samples. In this paper, we propose the variant-set mixed model association tests (SMMAT) for continuous and binary traits using the generalized linear mixed model framework. These tests can be applied to large-scale WGS studies involving samples with population structure and relatedness, such as in the National Heart, Lung, and Blood Institute's Trans-Omics for Precision Medicine (TOPMed) program. SMMATs share the same null model for different variant sets, and a virtue of this null model, which includes covariates only, is that it needs to be fit only once for all tests in each genome-wide analysis. Simulation studies show that all the proposed SMMATs correctly control type I error rates for both continuous and binary traits in the presence of population structure and relatedness. We also illustrate our tests in a real data example of analysis of plasma fibrinogen levels in the TOPMed program (n = 23,763), using the Analysis Commons, a cloud-based computing platform.
Recommended Citation
Chen, H., Huffman, J. E., Brody, J. A., Wang, C., Lee, S., Li, Z., Gogarten, S. M., Sofer, T., Bielak, L. F., Bis, J. C., Blangero, J., Bowler, R. P., Cade, B. E., Cho, M. H., Correa, A., Curran, J. E., de Vries, P. S., Glahn, D. C., Guo, X., Johnson, A. D., … Lin, X. (2019). Efficient Variant Set Mixed Model Association Tests for Continuous and Binary Traits in Large-Scale Whole-Genome Sequencing Studies. American journal of human genetics, 104(2), 260–274. https://doi.org/10.1016/j.ajhg.2018.12.012
Publication Title
Am J Hum Genet
DOI
10.1016/j.ajhg.2018.12.012
Academic Level
faculty
Mentor/PI Department
Office of Human Genetics
Comments
Copyright © 2018 American Society of Human Genetics.