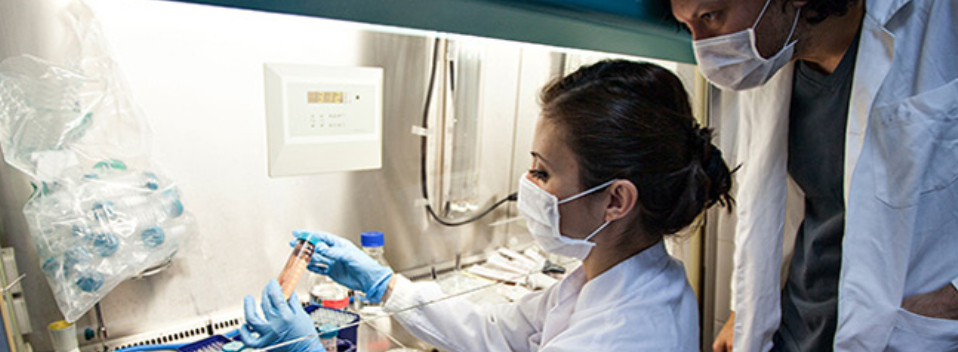
School of Medicine Publications and Presentations
Document Type
Article
Publication Date
7-2022
Abstract
Introduction: The novel coronavirus disease 2019 (COVID-19) has been a major health concern worldwide. This study aims to develop a Bayesian model to predict critical outcomes in patients with COVID-19.
Methods: Sensitivity and specificity were obtained from previous meta-analysis studies. The complex vulnerability index (IVC-COV2 index for its abbreviation in Spanish) was used to set the pretest probability. Likelihood ratios were integrated into a Fagan nomogram for posttest probabilities, and IVC-COV2 + National Early Warning Score (NEWS) values and CURB-65 scores were generated. Absolute and relative diagnostic gains (RDGs) were calculated based on pretest and posttest differences.
Results: The IVC-COV2 index was derived from a population of 1,055,746 individuals and was based on mortality in high-risk (71.97%), intermediate-risk (26.11%), and low-risk (1.91%) groups. The integration of models in which IVC-COV2 intermediate + NEWS ≥ 5 and CURB-65 > 2 led to a "number needed to (NNT) diagnose" that was slightly improved in the CURB-65 model (2 vs. 3). A comparison of diagnostic gains revealed that neither the positive likelihood ratio (P = 0.62) nor the negative likelihood ratio (P = 0.95) differed significantly between the IVC-COV2 NEWS model and the CURB-65 model.
Conclusion: According to the proposed mathematical model, the combination of the IVC-COV2 intermediate score and NEWS or CURB-65 score yields superior results and a greater predictive value for the severity of illness. To the best of our knowledge, this is the first population-based/mathematical model developed for use in COVID-19 critical care decision-making.
Recommended Citation
Baez, A. A., Lopez, O. J., Martinez, M., White, C., Ramirez-Slaibe, P., Martinez, L., & Castellanos, P. L. (2022). Assessment of a Comparative Bayesian-Enhanced Population-Based Decision Model for COVID-19 Critical Care Prediction in the Dominican Republic Social Security Affiliates. Cureus, 14(7), e26781. https://doi.org/10.7759/cureus.26781
Creative Commons License
This work is licensed under a Creative Commons Attribution 4.0 International License.
Publication Title
Cureus
DOI
10.7759/cureus.26781
Academic Level
faculty
Comments
Copyright © 2022, Baez et al.