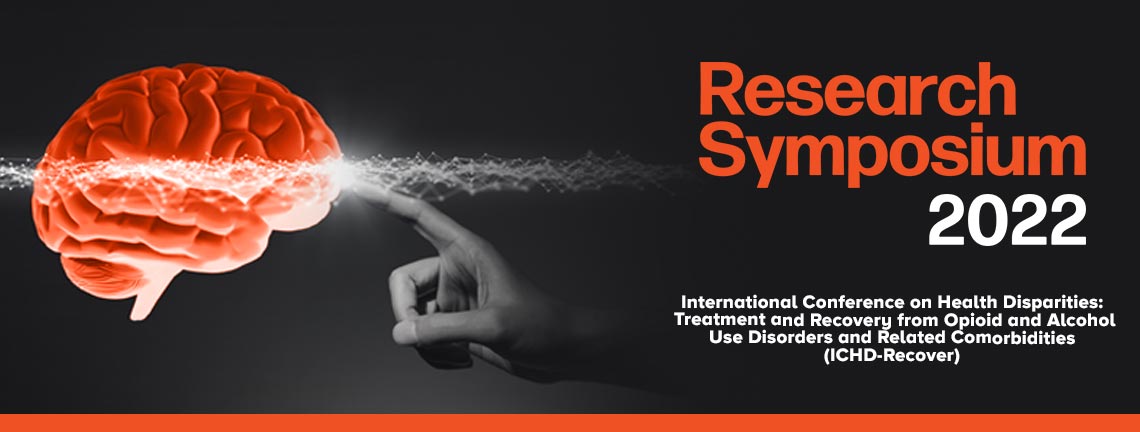
Posters
Presentation Type
Poster
Discipline Track
Biomedical ENGR/Technology/Computation
Abstract Type
Program Abstract
Abstract
Background: The textbook model of NNSV transcription predicts a gene expression gradient. However, multiple studies show non-gradient gene expression patterns or data inconsistent with a simple gradient. Regarding the latter, several studies show a dramatic decrease in gene expression over the last two genes of the respiratory syncytial virus (RSV) genome (a highly studied NNSV). The textbook model cannot explain these phenomena.
Methods: Computational models of RSV and vesicular stomatitis virus (VSV – another highly studied NNSV) transcription were written in the Python programming language using the Scientific Python Development Environment. The model code is freely available on GitHub: https://github.com/BCM-GCID/Publications/tree/main/Rethinking_NNSV_Gene_Expression. In brief, the models simulate one or more viral RNA-dependent RNA polymerases (pols) taking a random walk down a linear genome divided into chunks of a size approximating the footprint of a single pol and stochastically initiating and terminating transcription.
Results & Conclusions: Models incorporating ejective pol collisions and biased pol diffusion succeed in capturing published RSV and VSV gene expression patterns. Our model makes the following major predictions in need of wet lab experimental testing: 1) ejective collisions can occur between NNSV pols; 2) non-transcribing RSV pols undergo 5’ biased diffusion along the viral genome; and 3) an increase in the number of pols bound to and diffusing along an NNSV genome leads to more frequent pol-pol collisions and a sharper transcription gradient. Single molecule TIRF-based assays are needed to test predictions 1-2, while 3 can be tested using established minigenome or recombinant genome assays along with high throughput sequencing.
Recommended Citation
Piedra, Felipe-Andres, "Modeling nonsegmented negative-strand RNA virus (NNSV) transcription with ejective polymerase collisions and biased diffusion" (2023). Research Symposium. 36.
https://scholarworks.utrgv.edu/somrs/2022/posters/36
Included in
Biophysics Commons, Computational Biology Commons, Dynamic Systems Commons, Molecular Genetics Commons, Numerical Analysis and Computation Commons, Other Biochemistry, Biophysics, and Structural Biology Commons, Systems Biology Commons, Virology Commons
Modeling nonsegmented negative-strand RNA virus (NNSV) transcription with ejective polymerase collisions and biased diffusion
Background: The textbook model of NNSV transcription predicts a gene expression gradient. However, multiple studies show non-gradient gene expression patterns or data inconsistent with a simple gradient. Regarding the latter, several studies show a dramatic decrease in gene expression over the last two genes of the respiratory syncytial virus (RSV) genome (a highly studied NNSV). The textbook model cannot explain these phenomena.
Methods: Computational models of RSV and vesicular stomatitis virus (VSV – another highly studied NNSV) transcription were written in the Python programming language using the Scientific Python Development Environment. The model code is freely available on GitHub: https://github.com/BCM-GCID/Publications/tree/main/Rethinking_NNSV_Gene_Expression. In brief, the models simulate one or more viral RNA-dependent RNA polymerases (pols) taking a random walk down a linear genome divided into chunks of a size approximating the footprint of a single pol and stochastically initiating and terminating transcription.
Results & Conclusions: Models incorporating ejective pol collisions and biased pol diffusion succeed in capturing published RSV and VSV gene expression patterns. Our model makes the following major predictions in need of wet lab experimental testing: 1) ejective collisions can occur between NNSV pols; 2) non-transcribing RSV pols undergo 5’ biased diffusion along the viral genome; and 3) an increase in the number of pols bound to and diffusing along an NNSV genome leads to more frequent pol-pol collisions and a sharper transcription gradient. Single molecule TIRF-based assays are needed to test predictions 1-2, while 3 can be tested using established minigenome or recombinant genome assays along with high throughput sequencing.