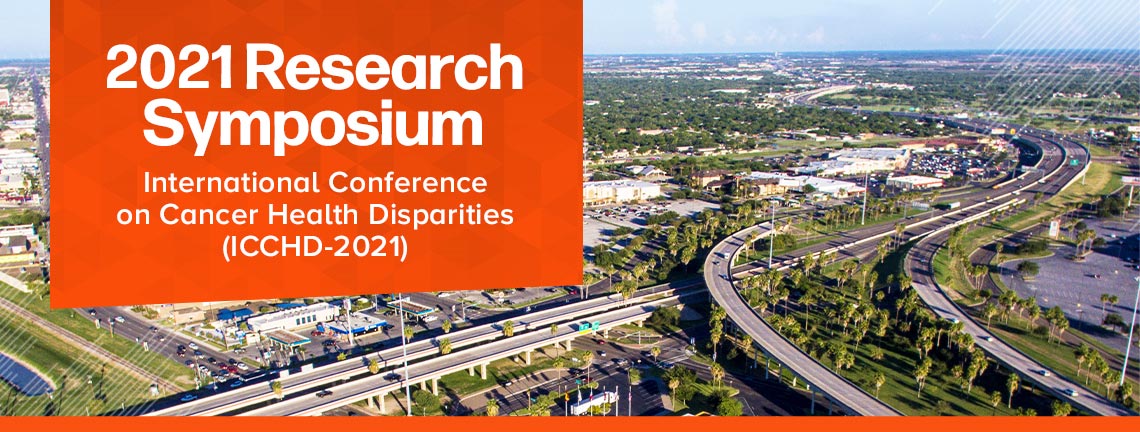
Talks
Academic/Professional Position (Other)
Computer Science
Presentation Type
Oral Presentation
Discipline Track
Biomedical ENGR/Technology/Computation
Abstract Type
Research/Clinical
Abstract
Background: Kinases are enzymes that have proven to be important drug targets due to their role in critical biological mechanisms such as phosphorylation. Phosphorylation happens when a kinase catalyzes the transfer of a phosphate group to a protein in a phosphorylated site, which then becomes known as the substrate of the kinase. Any dysregulation of protein phosphorylation causes a wide range of complex diseases including cancer. Thus, discovering the links between kinases and their substrates (i.e. predicting kinase-substrate associations (KSAs)) is crucial in developing effective and sustainable treatments. Presently, less than 5% of phosphorylated sites have an associated kinase, and existing prediction methods tend to favor well-known kinases. In this project, we use algorithms (NetREX) that were developed in the context of gene regulatory networks to create a network of kinases and substrates to improve prediction precision by uncovering hidden KSAs, while also comprehensively analyzing existing computational methods.
Methods: We modify NetRex, a method developed to find the links between transcription factors and genes, to create a network, where kinases and their phosphorylated sites are nodes and the known associations between them (KSAs) are represented by edges. We use the network component analysis model (NCA), which assumes that each kinase is characterized by its activity. NCA explains the phosphorylation of each phosphosite as a linear combination of the activities of its regulating kinases. We iteratively add and remove edges in the network, where co-phosphorylated sites are co-regulated, and kinases with correlated activities co-regulate the same site. Lastly, our edges are ranked by their confidence score, which is their impact on the overall performance of the linear model.
Results: Once we ran the algorithms on our data, we were able to obtain a network of kinases and substrates. We are currently in the process of performing cross validation to assess sensitivity and specificity of our network using methods such as 5-fold and leave-one-out. In addition, we will comprehensively analyze and compare our results with other computational methods to see if an improvement was achieved.
Conclusions: Identifying kinases causing abnormal phosphorylation is essential for drug discovery and effective treatment. Current computational prediction methods tend to find KSAs for kinases that already have several phosphorylated sites associated with them. Based on our results, we anticipate to uncover KSAs for kinases that do not have many phosphorylated sites associated with them priorly.
Recommended Citation
Irisson, Hasbanny and Ayati, Marzieh, "Precise Method to Identify Kinase Drug Targets in Complex Diseases: The First Step Towards Sustainable and Effective Treatment" (2023). Research Symposium. 39.
https://scholarworks.utrgv.edu/somrs/theme1/track1/39
Included in
Bioinformatics Commons, Biotechnology Commons, Data Science Commons, Medicine and Health Sciences Commons
Precise Method to Identify Kinase Drug Targets in Complex Diseases: The First Step Towards Sustainable and Effective Treatment
Background: Kinases are enzymes that have proven to be important drug targets due to their role in critical biological mechanisms such as phosphorylation. Phosphorylation happens when a kinase catalyzes the transfer of a phosphate group to a protein in a phosphorylated site, which then becomes known as the substrate of the kinase. Any dysregulation of protein phosphorylation causes a wide range of complex diseases including cancer. Thus, discovering the links between kinases and their substrates (i.e. predicting kinase-substrate associations (KSAs)) is crucial in developing effective and sustainable treatments. Presently, less than 5% of phosphorylated sites have an associated kinase, and existing prediction methods tend to favor well-known kinases. In this project, we use algorithms (NetREX) that were developed in the context of gene regulatory networks to create a network of kinases and substrates to improve prediction precision by uncovering hidden KSAs, while also comprehensively analyzing existing computational methods.
Methods: We modify NetRex, a method developed to find the links between transcription factors and genes, to create a network, where kinases and their phosphorylated sites are nodes and the known associations between them (KSAs) are represented by edges. We use the network component analysis model (NCA), which assumes that each kinase is characterized by its activity. NCA explains the phosphorylation of each phosphosite as a linear combination of the activities of its regulating kinases. We iteratively add and remove edges in the network, where co-phosphorylated sites are co-regulated, and kinases with correlated activities co-regulate the same site. Lastly, our edges are ranked by their confidence score, which is their impact on the overall performance of the linear model.
Results: Once we ran the algorithms on our data, we were able to obtain a network of kinases and substrates. We are currently in the process of performing cross validation to assess sensitivity and specificity of our network using methods such as 5-fold and leave-one-out. In addition, we will comprehensively analyze and compare our results with other computational methods to see if an improvement was achieved.
Conclusions: Identifying kinases causing abnormal phosphorylation is essential for drug discovery and effective treatment. Current computational prediction methods tend to find KSAs for kinases that already have several phosphorylated sites associated with them. Based on our results, we anticipate to uncover KSAs for kinases that do not have many phosphorylated sites associated with them priorly.