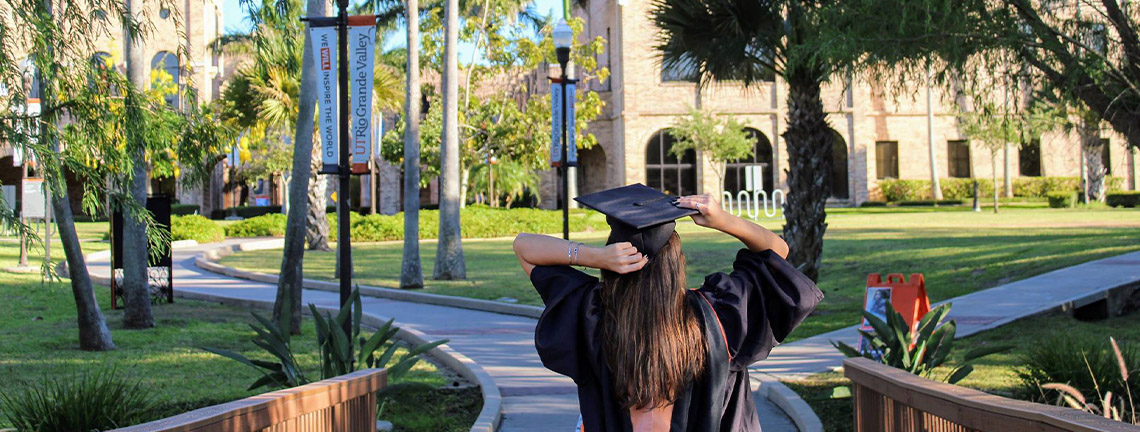
Theses and Dissertations
Date of Award
5-2024
Document Type
Thesis
Degree Name
Master of Science (MS)
Department
Applied Statistics and Data Science
First Advisor
Tamer Oraby
Second Advisor
Xuan Wang
Third Advisor
Rodrigo Hansapani
Abstract
Advancements in digitalization has yielded enormous growth of data on online platforms, overwhelming users with multitude of options to choose from. Recommender systems narrow down these options to a few relevant ones thereby facilitating the decision-making processes for users. This study presents a framework for integrating variational bias sampling into model-based collaborative filtering techniques for recommender systems. Variational bias sampling is a novel and unique way to account for random factors that affect explicit ratings in collaborative filtering recommender systems. A Gaussian distribution is used to model all the possible random factors that could affect ratings. Sampling user and item biases from this expansive distribution provides a more flexible and robust representation of user and item biases. In this framework, Matrix Factorization with variational bias sampling (MF_vbs) and Neural Collaborative Filtering with variational bias sampling (NCF_vbs) are introduced as the variational bias counterparts to the baseline Matrix Factorization and Neural Collaborative Filtering whiles Matrix Factorization with bias (MF_bs) and Neural Collaborative Filtering with bias (NCF_bs) represent the classical bias integration. Eight (8) real-life explicit rating datasets are used to evaluate the performance of the models using coefficient of determination (R2), F1Score, and Normalized Discounted Cumulative Gain (NDCG). The results of this extensive comparison shows NCF_vbs is the overall best performing method followed by MF_vbs. Additionally, accounting for biases via the classical approach also improves performance but not as much as variational bias sampling. In summary, variational bias sampling presents a promising solution to account for random factors that influence ratings, thereby offering improved accuracy and personalized recommendation systems.
Recommended Citation
Stephens, P. (2024). Variational Bias Sampling for Collaborative Filtering Recommender Systems [Master's thesis, The University of Texas Rio Grande Valley]. ScholarWorks @ UTRGV. https://scholarworks.utrgv.edu/etd/1519
Comments
Copyright 2024 Prisca Stephens.
https://go.openathens.net/redirector/utrgv.edu?url=https://www.proquest.com/pqdtglobal1/dissertations-theses/variational-bias-sampling-collaborative-filtering/docview/3085293074/sem-2?accountid=7119