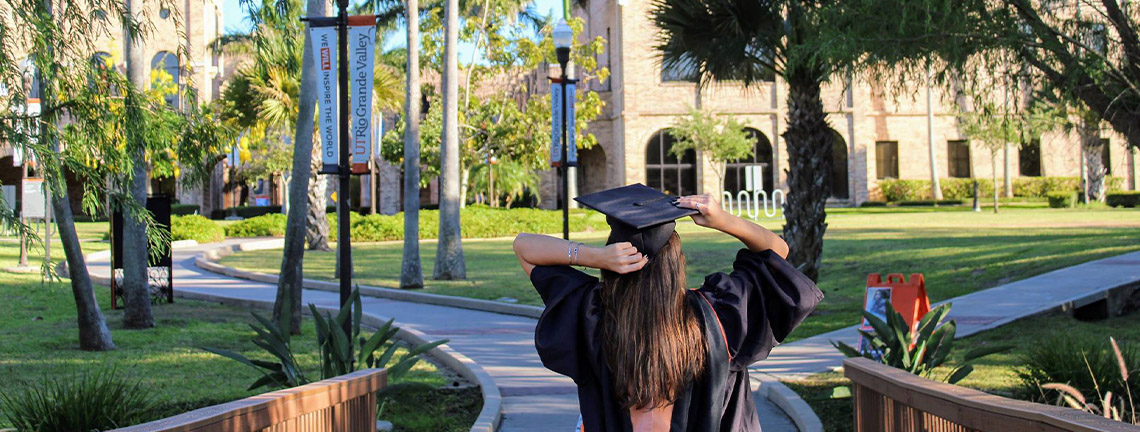
Theses and Dissertations
Date of Award
5-2022
Document Type
Thesis
Degree Name
Master of Science (MS)
Department
Civil Engineering
First Advisor
Dr. Jungseok Ho
Second Advisor
Dr. Dongchul Kim
Third Advisor
Dr. Fatemeh Nazari
Abstract
Salinity is an important metric in the Laguna Madre for establishing the long term health of the local ecological population. By utilizing Deep Learning (DL) techniques, the predicted and forecasted salinity in the Laguna Madre is generated from data provided by the Moderate Resolution Imaging Spectroradiometer (MODIS)-Aqua satellite.
Currently, only one other DL model has been used to forecast Sea Surface Salinity (SSS), being a Recurrent Neural Network (RNN). However, the RNN model requires the prediction of a full area of salinity to function.
As such, several model architectures were tested, with the best one, being a Multi-input MPNN, utilized to evaluate the feasibility of forecasting utilizing simpler DL models. The results show that a one-day forecast is plausible, while three and five-day forecasts would require a data-rich environment, unlike that of the Laguna Madre.
Recommended Citation
Flores, Martin J. Jr., "Forecasting Salinity in the Laguna Madre Using Deep Learning" (2022). Theses and Dissertations. 1039.
https://scholarworks.utrgv.edu/etd/1039
Comments
Copyright 2022 Martin J. Flores Jr. All Rights Reserved.
https://go.openathens.net/redirector/utrgv.edu?url=https://www.proquest.com/dissertations-theses/forecasting-salinity-laguna-madre-using-deep/docview/2707899424/se-2?accountid=7119