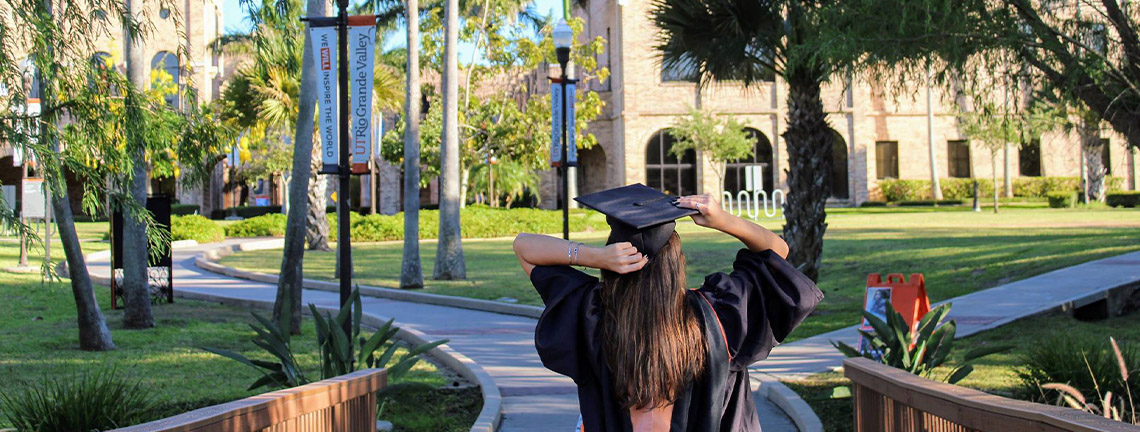
Theses and Dissertations
Date of Award
5-2022
Document Type
Thesis
Degree Name
Master of Science (MS)
Department
Manufacturing Engineering
First Advisor
Dr. Douglas Timmer
Second Advisor
Dr. Jianzhi Li
Third Advisor
Dr. Hiram Moya
Abstract
The electric load industry has seen a significant transformation over the last few decades, culminating in the establishment and implementation of electricity markets. This transition separates electric generation services into a distinct, more competitive sector of the industry, allowing for the introduction of greater unpredictability into the system. Forecasting power system load has developed into a core research area in power and energy demand engineering in order to maintain a constant balance between electricity supply and demand. The purpose of this thesis dissertation is to reduce power system uncertainty by improving forecasting accuracy through the use of sophisticated machine learning techniques. Additionally, this research provides sophisticated machine learning-based forecasting methodologies for the three forecasting professions from a variety of perspectives, incorporating several advanced deep learning features such as Naïve/default, Hyperparameter Tuning, and Custom Early Stopping. We begin by creating long-term memory (LSTM) and gated recurrent unit (GRU) models for ERCOT demand data, and then compare them to some of the most well-known supervised machine learning models, such as ARIMA and SARIMA, to identify the best set of models for long- and short-term load forecasting. We will also use multiple comparison approaches, such as the radar chart and the Pygal radar chart, to perform a thorough evaluation of each of the deep learning models before settling on the best model.
Recommended Citation
Hossain, Md Riyad, "Machine Learning Tools in the Predictive Analysis of ERCOT Load Demand Data" (2022). Theses and Dissertations. 1056.
https://scholarworks.utrgv.edu/etd/1056
Comments
Copyright 2022 Md Riyad Hossain. All Rights Reserved.
https://go.openathens.net/redirector/utrgv.edu?url=https://www.proquest.com/dissertations-theses/machine-learning-tools-predictive-analysis-ercot/docview/2698666398/se-2?accountid=7119