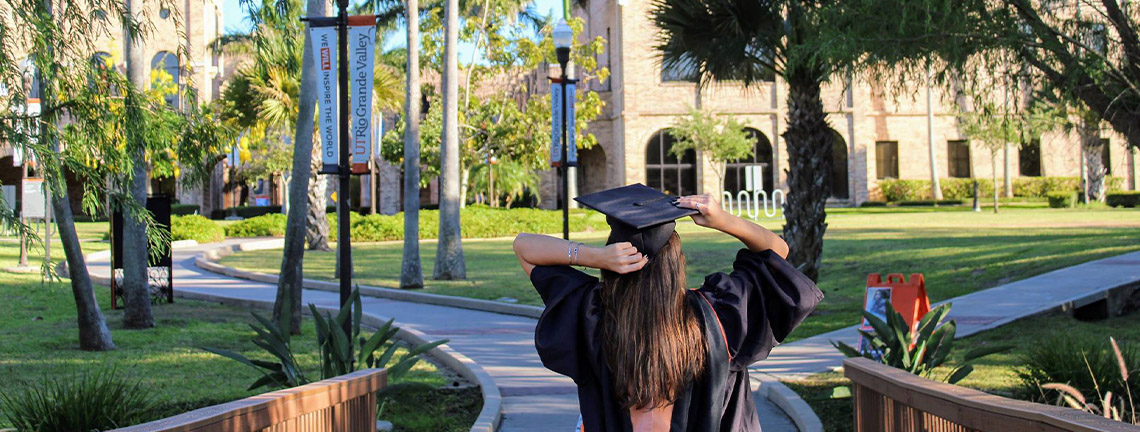
Theses and Dissertations
Date of Award
5-2022
Document Type
Thesis
Degree Name
Master of Science (MS)
Department
Electrical Engineering
First Advisor
Dr. Mostafizur Rahman
Second Advisor
Dr. Mark Chu
Third Advisor
Dr. Nantakan Wongkasem
Abstract
Due to advancements in data acquisition techniques, collecting datasets representing samples from multi-views has become more common recently (Jia et al. 2019). For instance, in genomics, a lymphoma patient’s dataset may include data on gene expression, single nucleotide polymorphism (SNP), and array Comparative genomic hybridization (aCGH) measurements. Learning from multiple views about the same objective, in general, obtains a better understanding of the hidden patterns of the data compared to learning from a single view data. Most of the existing multi-view learning techniques such as canonical correlation analysis (Hotelling et al. 1936) and multi-view support vector machine (Farquhar et al. 2006), multiple kernel learning (Zhang et al. 2016) are focused on extracting the shared information among multiple datasets.
However, in some real-world applications, it’s appealing to extract the discriminative knowledge of multiple datasets, namely discriminative data analytics. For example, consider the one dataset as gene-expression measurements of cancer patients, and the other dataset as the gene-expression levels of healthy volunteers and the goal is to cluster cancer patients according to the molecular sub-types. Performing a single view analysis such as principal component analysis (PCA) on any of the dataset yields information related to the common knowledge between the two datasets (Garte et al. 1996). Addressing such challenge, contrastive PCA (Abid et al. 2017) and discriminative (d) PCA in (Jia et al. 2019) are proposed in to extract one dataset-specific information often missed by PCA.
Inspired by dPCA, we propose a novel discriminative multi-view learning algorithm, namely Non-negative Discriminative Analysis (DNA), to extract the unique information of one dataset (a.k.a. view) with respect to the other dataset. This boils down to solving a non-negative matrix factorization problem. Furthermore, we apply the proposed DNA framework in various real-world down-stream machine learning applications such as feature selections, dimensionality reduction, classification, and clustering.
Recommended Citation
Kaish, Md Imrul, "Non-Negative Discriminative Data Analytics" (2022). Theses and Dissertations. 1059.
https://scholarworks.utrgv.edu/etd/1059
Comments
Copyright 2022 Md Imrul Kaish. All Rights Reserved.
https://go.openathens.net/redirector/utrgv.edu?url=https://www.proquest.com/dissertations-theses/non-negative-discriminative-data-analytics/docview/2699732019/se-2?accountid=7119