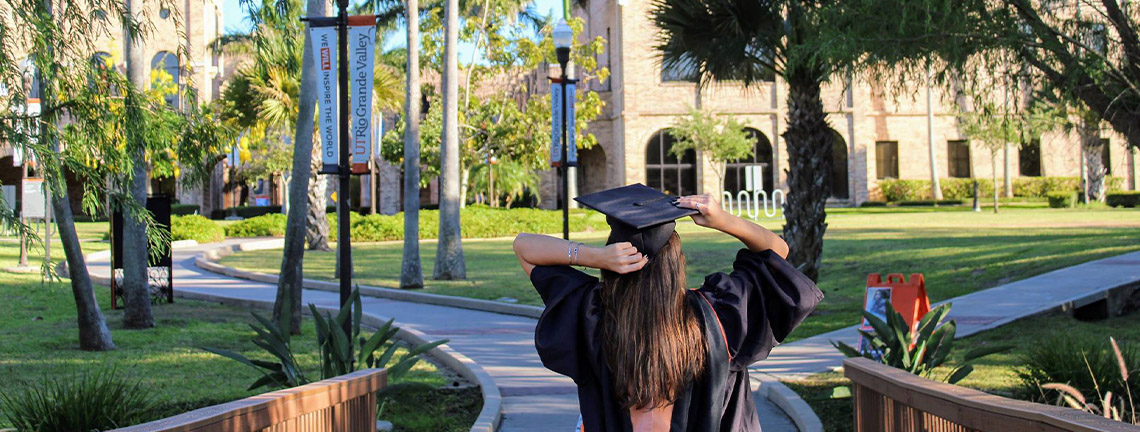
Theses and Dissertations
Date of Award
7-2022
Document Type
Thesis
Degree Name
Master of Science (MS)
Department
Mechanical Engineering
First Advisor
Dr. Constantine Tarawneh
Second Advisor
Dr. Mohamadhossein Noruzoliaee
Third Advisor
Dr. Fatemeh Nazari
Abstract
By continuously monitoring train bearing health in terms of temperature and vibration levels of bearings tested in a laboratory setting, statistical regression models have been developed to establish relationships between the sensor-acquired bearing health data with several explanatory factors that potentially influence the bearing deterioration. Despite their merits, statistical models fall short of reliable prediction accuracy levels since they entail restrictive assumptions, such as a priori known functional relationship between the response and input variables. A data-driven machine learning algorithm is presented, which can unravel the nonlinear deterioration model purely based on the bearing health data, even when the structure is not apparent. More specifically, a Gradient Boosting Machine is trained using vast amounts of laboratory data collected over the course of over a decade. This will help predict bearing failure, thus, providing railroads and railcar owners the opportunity to schedule preventive maintenance cycles rather than costly reactive ones.
Recommended Citation
Villafranca, Leonel, "Predicting the Remaining Service Life of Railroad Bearings: Leveraging Machine Learning and Onboard Sensor Data" (2022). Theses and Dissertations. 1111.
https://scholarworks.utrgv.edu/etd/1111
Comments
Copyright 2022 Leonel Villafranca. All Rights Reserved.
https://go.openathens.net/redirector/utrgv.edu?url=https://www.proquest.com/dissertations-theses/predicting-remaining-service-life-railroad/docview/2739757613/se-2?accountid=7119