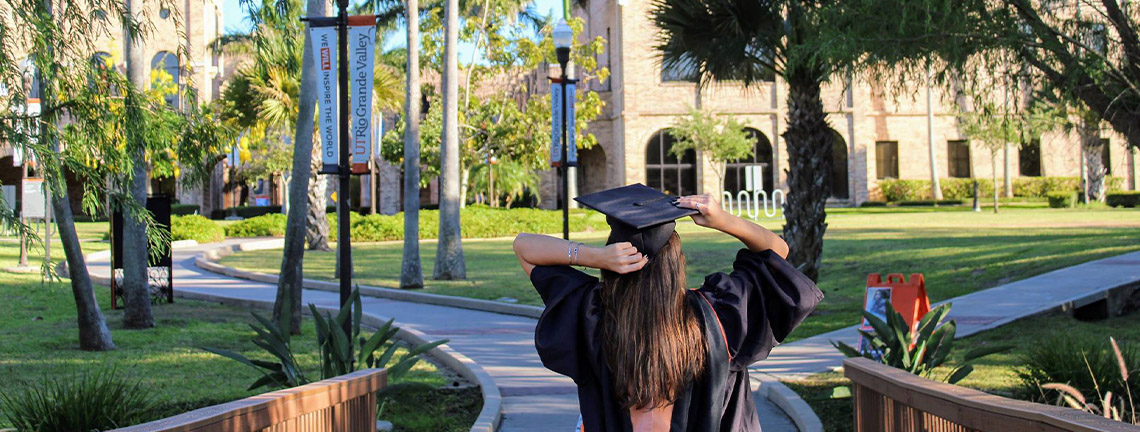
Theses and Dissertations
Date of Award
12-2022
Document Type
Thesis
Degree Name
Master of Science (MS)
Department
Computer Science
First Advisor
Dr. Dongchul Kim
Second Advisor
Dr. Erik Enriquez
Third Advisor
Dr. Emmett Tomai
Abstract
Despite advances in drug research and development, there are few and ineffective treatments for a variety of diseases. Virtual screening can drastically reduce costs and accelerate the drug discovery process. Binding site identification is one of the initial and most important steps in structure-based virtual screening. Identifying and defining protein cavities that are likely to bind to a small compound is the objective of this task. In this research, we propose four different convolutional neural networks for predicting ligand-binding sites in proteins. A parallel optimized data pipeline is created to enable faster training of these neural network models on minimal hardware. The effectiveness of each method is assessed on well-established ligand binding site datasets. It is then compared with the state-of-the-art and widely used methods for ligand binding site identification. The result shows that our methods outperform most of the other methods and are comparable to the state-of-the-art methods.
Recommended Citation
Islam, Md Ashraful, "AtomLbs: An Atom Based Convolutional Neural Network for Druggable Ligand Binding Site Prediction" (2022). Theses and Dissertations. 1149.
https://scholarworks.utrgv.edu/etd/1149
Comments
Copyright 2022 Md Ashraful Islam. All Rights Reserved.
https://go.openathens.net/redirector/utrgv.edu?url=https://www.proquest.com/dissertations-theses/atomlbs-atom-based-convolutional-neural-network/docview/2802168089/se-2?accountid=7119