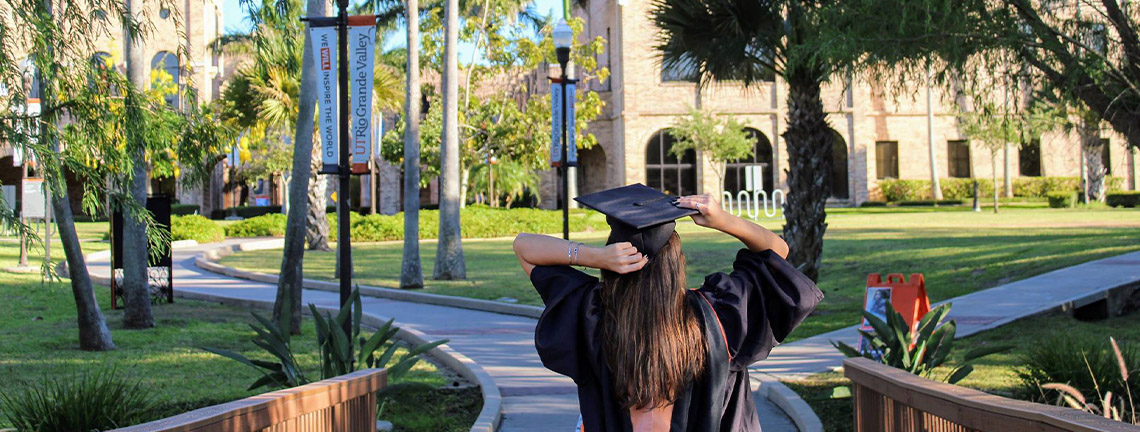
Theses and Dissertations
Date of Award
5-2023
Document Type
Thesis
Degree Name
Master of Science (MS)
Department
Applied Statistics and Data Science
First Advisor
Dr. Tamer Oraby
Second Advisor
Dr. George Yanev
Third Advisor
Dr. Hansapani Rodrigo
Abstract
Chronic kidney disease (CKD) is a significant complication that contributes to diabetes-related mortality in the United States, and there is growing evidence that sodium-glucose cotransporter 2 inhibitors (SGLT2i) can slow its progression. However, observational studies may suffer from confounding by indication, where patient characteristics and disease severity influence the decision to prescribe SGLT2i. This study utilized electronic health records of individuals with diabetes (from TriNetX) to investigate the effectiveness of SGLT2i on CKD progression. The database provided detailed information on patients’ CKD status, demographics, diagnosis, procedures, and medications, along with corresponding dates of diagnosis and prescription. The study comprised of 38,776 patients aged 18 years and above with 1 (or >) year history of diabetes who initiated treatment with SGLT2i between May 9, 2013, and July 7, 2021. To address potential confounding by indication in observational studies, we utilized propensity score matching. We also addressed the issue of imbalanced classification in medical datasets by applying balanced bagging (with bootstrap aggregation) and Synthetic Minority Oversampling Technique (SMOTE). By overcoming the underrepresented minority class and reducing bias in the machine learning (ML) models, we ensured accurate causal identification of CKD prevalence by employing the following ML models: logistic regression, decision tree, random forest, extreme gradient boosting, support vector classifier and artificial neural network. Our results suggest that SGLT2i have a protective effect on CKD outcomes, providing valuable insights into the practical efficacy of this treatment and potentially serving as a clinical decision-making tool. Our findings have significant clinical implications for the healthcare sector and suggest that these techniques can improve the reliability of ML methods.
Recommended Citation
Eshun, Solomon, "A Machine Learning Approach to Evaluate the Effect of Sodium-Glucose Cotransporter-2 Inhibitors on Chronic Kidney Disease in Diabetes Patients" (2023). Theses and Dissertations. 1211.
https://scholarworks.utrgv.edu/etd/1211
Comments
Copyright 2023 Solomon Eshun. All Rights Reserved.
https://go.openathens.net/redirector/utrgv.edu?url=https://www.proquest.com/dissertations-theses/machine-learning-approach-evaluate-effect-sodium/docview/2842776574/se-2?accountid=7119