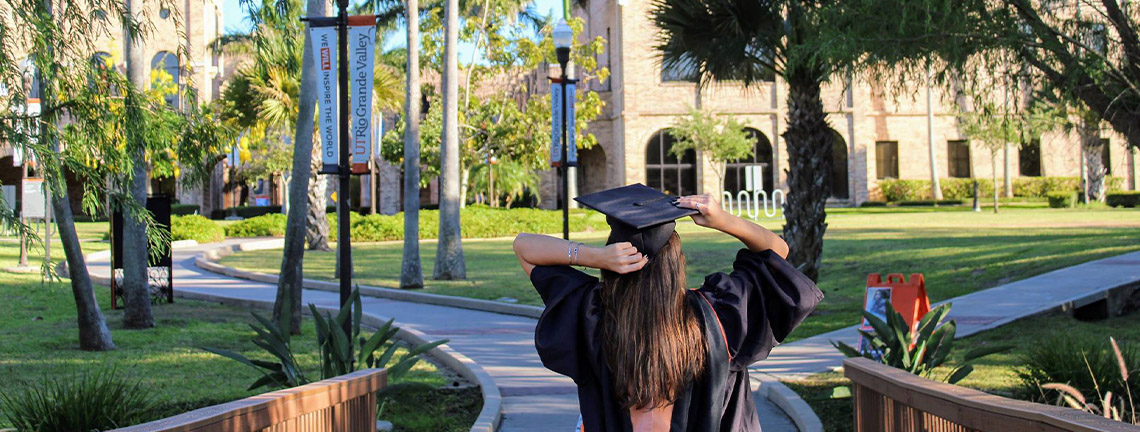
Theses and Dissertations
Effects of Missing Data Imputation Methods on Univariate Time Series Forecasting with Arima and LSTM
Date of Award
5-2023
Document Type
Thesis
Degree Name
Master of Science (MS)
Department
Mathematics
First Advisor
Dr. Kristina Vatcheva
Second Advisor
Dr. Oleg Musin
Third Advisor
Dr. Santanu Chakraborty
Abstract
Missing data are common in real-life studies and missing observations within the univariate time series cause analytical problems in the flow of the analysis. Imputation of missing values is an inevitable step in the analysis of every incomplete univariate time series data. The reviewed literature has shown that the focus of existing studies is on comparing the distribution of imputed data. There is a gap of knowledge on how different imputation methods for univariate time series data affect the fit and prediction performance of time series models. In this work, we evaluated the predictive performance of autoregressive integrated moving average (ARIMA) and long short-term memory (LSTM) models on imputed time-series data using Kalman smoothing on ARIMA, Kalman smoothing on structural time series model, mean imputation, exponentially weighted moving average, simple moving average, linear, cubic spline, stine, and KNN interpolation techniques under missing completely at random (MCAR) mechanism. Missing values were generated at 10%, 15%, 25%, and 35% rates using complete data of 24-hour ambulatory diastolic blood pressure readings. The performance of models was compared on imputed and original data using mean absolute percentage error (MAPE) and root mean square error (RMSE). Kalman smoothing on structural time series, exponentially weighted moving average, and Kalman smoothing on ARIMA were the best missing data replacement techniques as the gap of the missingness increased. The performance of mean imputation, cubic spline, KNN, and the other simple interpolation methods reduced significantly as the gap of missingness increased. The LSTM gave better predictions on the original training data, but the ARIMA predictions on imputed data gave consistent results across the four scenarios.
Recommended Citation
Niako, Nicholas, "Effects of Missing Data Imputation Methods on Univariate Time Series Forecasting with Arima and LSTM" (2023). Theses and Dissertations. 1244.
https://scholarworks.utrgv.edu/etd/1244
Comments
Copyright 2023 Nicholas Niako. All Rights Reserved.
https://go.openathens.net/redirector/utrgv.edu?url=https://www.proquest.com/dissertations-theses/effects-missing-data-imputation-methods-on/docview/2842775092/se-2?accountid=7119