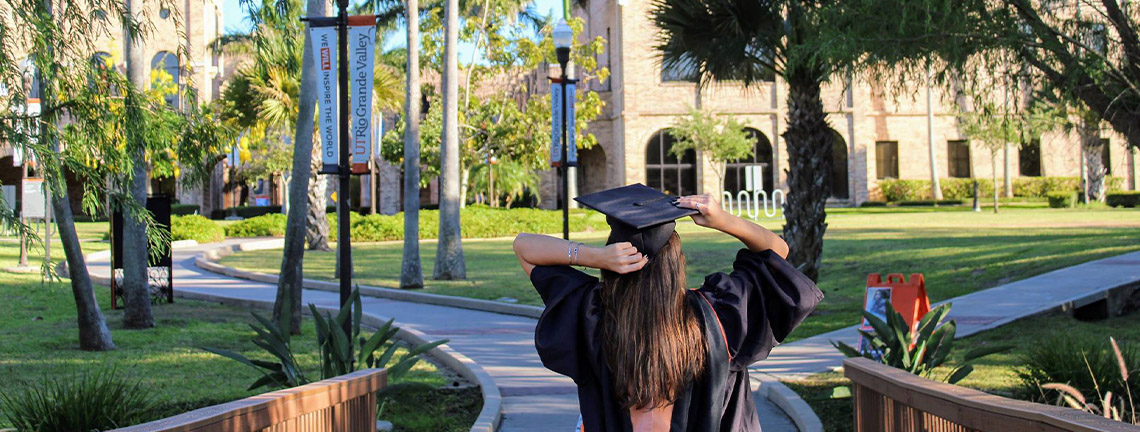
Theses and Dissertations
Date of Award
5-2023
Document Type
Thesis
Degree Name
Master of Science (MS)
Department
Mechanical Engineering
First Advisor
Dr. Constantine Tarawneh
Second Advisor
Dr. Tohid Sardarmehni
Third Advisor
Dr. Horacio Vasquez
Abstract
This paper presents a novel control method for quadcopters that achieves near-optimal tracking control for input-affine nonlinear quadcopter dynamics. The method uses a reinforcement learning algorithm called Single Network Adaptive Critics (SNAC), which approximates a solution to the discrete-time Hamilton-Jacobi-Bellman (DT-HJB) equation using a single neural network trained offline. The control method involves two SNAC controllers, with the outer loop controlling the linear position and velocities (position control) and the inner loop controlling the angular position and velocities (attitude control). The resulting quadcopter controller provides optimal feedback control and tracks a trajectory for an infinite-horizon, and it is compared with commercial optimal control software. Furthermore, the closed-loop controller can control the system with any initial conditions within the domain of training. Overall, this research demonstrates the benefits of using SNAC for nonlinear control, showing its ability to achieve near-optimal tracking control while reducing computational complexity. This paper provides insights into a new approach for controlling quadcopters, with potential applications in various fields such as aerial surveillance, delivery, and search and rescue.
Recommended Citation
Velazquez-Estrada, Alberto, "Near-Optimal Control of a Quadcopter Using Reinforcement Learning" (2023). Theses and Dissertations. 1267.
https://scholarworks.utrgv.edu/etd/1267
Comments
Copyright 2023 Alberto Velazquez-Estrada. All Rights Reserved.
https://go.openathens.net/redirector/utrgv.edu?url=https://www.proquest.com/dissertations-theses/near-optimal-control-quadcopter-using/docview/2842764435/se-2?accountid=7119