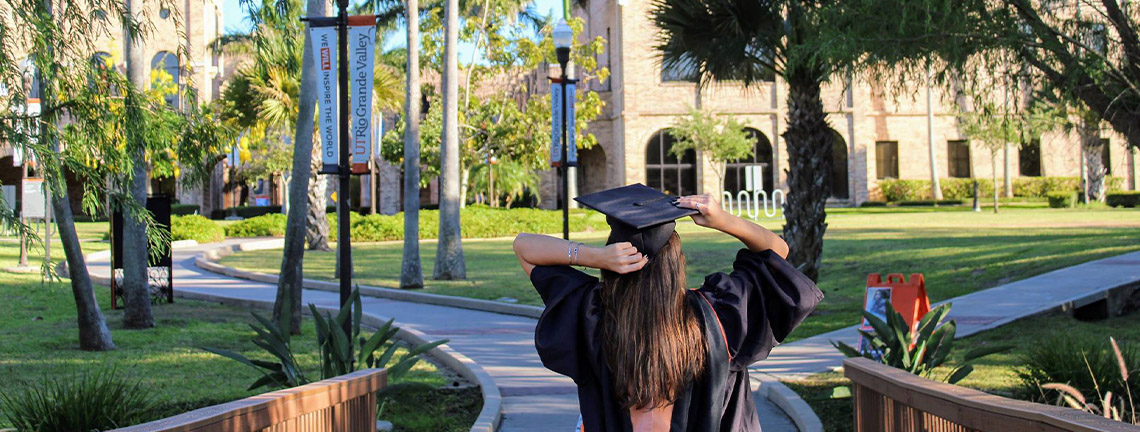
Theses and Dissertations
Date of Award
8-2022
Document Type
Thesis
Degree Name
Master of Science (MS)
Department
Applied Statistics and Data Science
First Advisor
Dr. Hansapani Rodrigo
Second Advisor
Dr. Tamer Oraby
Third Advisor
Dr. Erwin Suazo
Abstract
Influenced by the seminal work, “Physics Informed Neural Networks” by Raissi et al., 2017, there has been a growing interest in solving and parameter estimation of Nonlinear Partial Differential Equations (PDE) with Deep Neural networks in recent years. In fact, this has broadened the pathways and shed light on deep learning of stochastic differential equations (SDE) and stochastic PDE’s (SPDE).In this work, we intend to investigate the current approaches of solving and parameter estimation of the SDE/SPDE with deep neural networks and the possibility of extending them to obtain more accurate/stable solutions with residual systems and/or generative adversarial neural networks. We will also apply these methods to study real-world applications of SDE/SPDE problems. The combination of methods can improve speeds, accuracy, and lessen data-related difficulties in solving Stochastic PDEs. Such improvements can assist a wide array of the sciences in computational research and data sciences.
Recommended Citation
Flores, Stephanie L., "Neural Networks and Stochastic Differential Equations" (2022). Theses and Dissertations. 1272.
https://scholarworks.utrgv.edu/etd/1272
Comments
Copyright 2022 Stephanie L. Flores. All Rights Reserved.
https://go.openathens.net/redirector/utrgv.edu?url=https://www.proquest.com/dissertations-theses/neural-networks-stochastic-differential-equations/docview/2748416538/se-2?accountid=7119