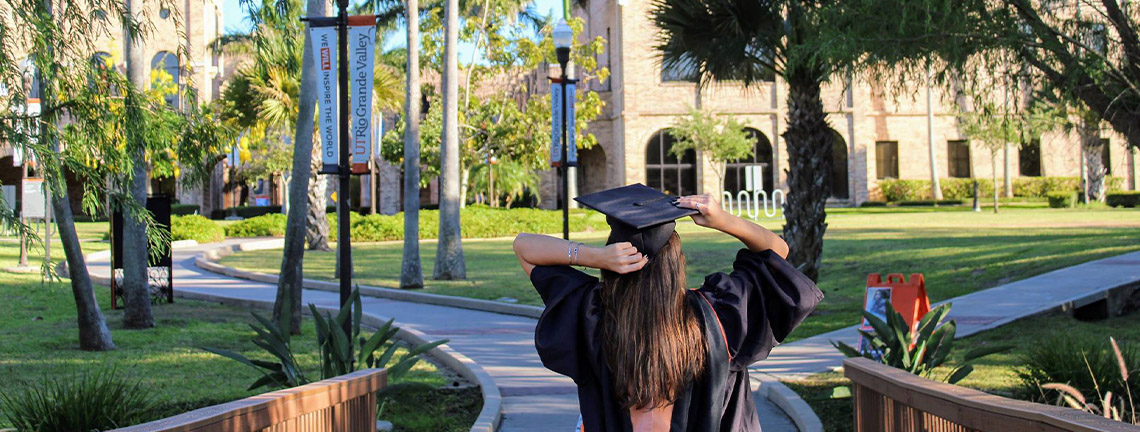
Theses and Dissertations
Date of Award
7-2023
Document Type
Thesis
Degree Name
Master of Science (MS)
Department
Physics
First Advisor
Soma Mukherjee
Second Advisor
Soumya Mohanty
Third Advisor
Malik Rakhmanov
Abstract
Core-Collapse Supernova (CCSN) is one of the most anticipated sources of Gravitational Waves (GW) arriving at the advanced LIGO detectors during the fourth observation run (O4). CCSN are rare, weak and unmodeled having a very low rate of occurrence in our galaxy (estimated 2 per century). Thus, detection of GW from CCSN is a challenging problem. An analysis pipeline used in this study is Multi-Layer Signal Enhancement with cWB and CNN or MuLaSEcC that combines Machine Learning methods with a network of Gravitational Wave detectors to identify and reconstruct signals from core collapse supernovae, while minimizing false alarms through the use of a trained convolutional neural network. The selection of the CCSN waveforms is based on principal component analysis followed by a K-means classification in a multi-variate parameter space. We have used a Convolutional Neural Network (CNN) to train on 11 different simulated CCSN signals and evaluated its performance using four distinct non-rotating waveforms: Andresen_2016_s27, Andresen_2016_s11, mesa20_pert, and Mezzacappa_2020_D15-3D.The analysis yielded improved results in terms of reduction in the false alarm rate and also produced higher detection efficiency as a function of Signal to noise ratio(SNR). The parameter range of progenitor mass, peak frequency, and duration for the four unknown supernova signals is correctly estimated and the pipeline has reconstructed the unknown waveforms with high degree of accuracy over a very large bandwidth.
Recommended Citation
Sedhai, B. (2023). A Convolutional Neural Network Based Approach to Study the Gravitational Waves From Core-Collapse Supernovae in Ligo's Third Observation Run: Detection Efficiency and Parameter Estimation [Master's thesis, The University of Texas Rio Grande Valley]. ScholarWorks @ UTRGV. https://scholarworks.utrgv.edu/etd/1276
Comments
Copyright 2023 Bhawana Sedhai. All Rights Reserved.
https://go.openathens.net/redirector/utrgv.edu?url=https://www.proquest.com/dissertations-theses/convolutional-neural-network-based-approach-study/docview/2861979542/se-2?accountid=7119