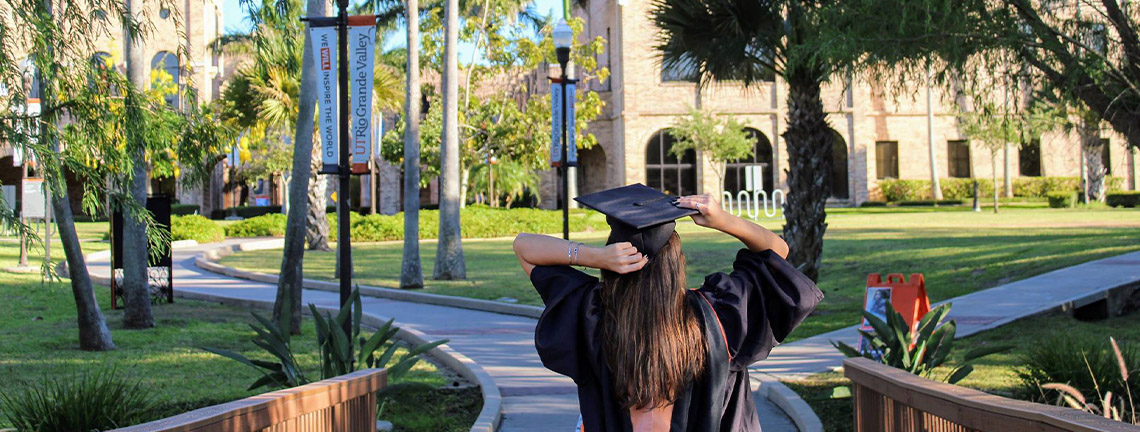
Theses and Dissertations
Date of Award
7-2023
Document Type
Thesis
Degree Name
Master of Science in Engineering (MSE)
Department
Civil Engineering
First Advisor
Mohsen Amjadian
Second Advisor
Philip Park
Third Advisor
Mohamadhossein Noruzoliaee
Abstract
Open-Deck (OD) steel truss railroad bridges are one of the most common types of railroad bridges in the United States. They are, however, significantly vulnerable to the dynamic effects of moving train load. In the long run, these dynamic effects cause fatigue damage to the structural members. The damage in most of the railroad bridges are monitored by visual inspections which are sometimes unreliable and inconsistent due to human error. This study explores the identification and classification of damage to an open deck railroad bridge from both time-domain (statistical features) and time-frequency domain features (Hilbert-Huang Transform) extracted through acceleration response using Machine Learning Classifier in MATLAB R2022a. A 3D finite element model of the bridge was created in SAP2000 and validated with the field-testing data. The changes in acceleration time-history responses obtained under the different damage cases are utilized to detect damage using two Machine Learning Classifiers: Support Vector Machine (SVM) and K-Nearest Neighbor (KNN) algorithms. The extracted features are used to train and cross validate the algorithm and indicated high level of precision (more than 95%) in identifying and classifying the damage in the bridge and more than 85% in identifying defect in the rail.
Recommended Citation
Pokhrel, M. (2023). A Data-Driven Method for Damage Detection in an Open Deck Steel Truss Railroad Bridge Under the Moving Train Load [Master's thesis, The University of Texas Rio Grande Valley]. ScholarWorks @ UTRGV. https://scholarworks.utrgv.edu/etd/1277
Comments
Copyright 2023 Mahesh Pokhrel. All Rights Reserved.
https://go.openathens.net/redirector/utrgv.edu?url=https://www.proquest.com/dissertations-theses/data-driven-method-damage-detection-open-deck/docview/2862129956/se-2?accountid=7119