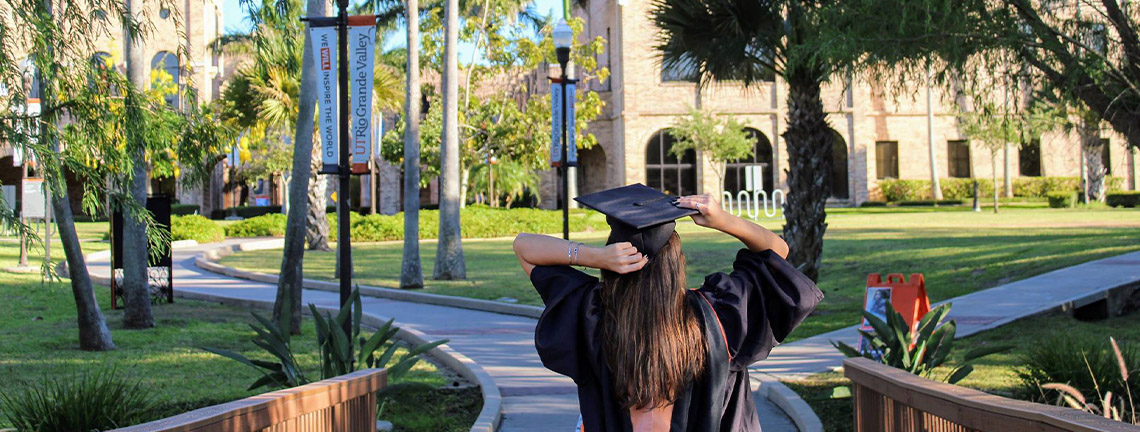
Theses and Dissertations
Date of Award
7-2023
Document Type
Thesis
Degree Name
Master of Science (MS)
Department
Physics
First Advisor
Soma Mukherjee
Second Advisor
Malik Rakhmanov
Third Advisor
Soumya D. Mohanty
Abstract
Core collapse supernovae (CCSN) are highly anticipated sources of gravitational waves during the fourth observation run (O4). CCSN signals are weak and unmodeled and the rate of occurrence in our galaxy is very low. Because of this, they provide a greater challenge to detect than previously detected GW sources. CCSN simulations are used to test the detection pipeline in the event a CCSN is detected. CCSN GW signals are often indistinguishable from the noise sources present in GW data. We present a multi layered signal enhancement pipeline which we have applied Machine Learning (ML) techniques. We have used a Convolutional Neural Network (CNN) to train on 10 different simulated CCSN signals, and then tested the preformance of our pipeline on rotating CCSN signals.
Recommended Citation
Benjamin, Michael Gale, "Analyzing the on Source Window of Supernova SN2019EJJ With a Multi Layered Signal Enhancement Algorithm With Coherent Waveburst and a Convolutional Neural Network" (2023). Theses and Dissertations. 1285.
https://scholarworks.utrgv.edu/etd/1285
Comments
Copyright 2023 Michael Gale Benjamin. All Rights Reserved.
https://go.openathens.net/redirector/utrgv.edu?url=https://www.proquest.com/dissertations-theses/analyzing-on-source-window-supernova-sn2019ejj/docview/2861609648/se-2?accountid=7119